2024 November - What I Have Read
Substack
Microsoft: Capacity Constrained - App Economy Insights [Link]
Highlight what to watch looking forward: 1) Microsoft is addressing its data centers’ increasing power demands by turning to nuclear energy, 2) Microsoft is launching autonomous AI agents in November, introducing tools that enable businesses to automate routine tasks and boosting efficiency: Copilot Studio allows business create their own AI agents with minimal coding knowledge; and it will offer 10 ready-to-use agents covering everyday business needs.
Can Large Language Models Reason? - AI: A Guide for Thinking Humans [Link]
Current evidence suggests that LLMs simulate reasoning rather than genuinely reasoning. This highlights the need for careful evaluation of LLMs’ generalization capabilities, especially as AI is increasingly integrated into complex decision-making contexts.
Meta’s early AR unveiling may come with competitive trade-offs. According to Bloomberg, Apple has launched its own smart glasses initiative, a market study called “Atlas,” signaling a potential shift from its high-end \(\$3,500\) Vision Pro VR headset. Apple recently cut its Vision Pro shipment target to less than half a million units in the first year—down from an initial target of 3 million.
Meta is pursuing a two-pronged approach to AR glasses:
- Orion has a hardware challenge (powerful but still cumbersome).
- Rayban Meta glasses have a software challenge (lightweight but only offering relatively simple use cases).
― Meta: AI Killed The Video Star - App Economy Insights [Link]
Current stage of Meta Orion: 1) prototype (not product), 2) advanced AR display (micro LED projectors and silicon carbide lenses), 3) interactive AI capabilities, 4) hardware complexity (neural wristband for control and a wireless compute puck for functionality), 5) high costs ($10K per unit) and limited production, 6) future vision - to release a consumer-ready AR device within a few years, targeting a more affordable product closer to smartphone price levels.
AI’s impact on Meta: 1) engagement: Meta’s recommendation system provides most relevant content to users, attracting users to spend more time on Apps, 2) monetization: Gen AI assists with ad copy, image, and video production, while new models analyze user actions before serving specific ads, ultimately increasing conversions at the margins.
About Meta AI Studio (for developers to create, train, and deploy custom AI models across Meta’s ecosystem): the goal is to drive the next wave of consumer apps and maximize ad potential across its platforms.
The discussion on “The Death of Creator Economy” is interesting and insightful. It’s true - as Meta moves towards an AI-centered model, creators may find themselves competing against the platforms that once supported them. By relying on AI, Meta could optimize ad placements and user engagement without the cost of creator compensation. This is a departure from platforms like YouTube, which incentivize creators with ad revenue shares. The broader impact could reshape the landscape of online content. As AI-generated feeds become the norm, audiences may eventually consume content that’s been strategically tailored by algorithms rather than creators. The creative autonomy that once defined social media could shift to a more managed, homogenized experience, where what we see is driven less by personal expression and more by AI-calculated engagement metrics.
Pichai discussed five ways customers use Cloud:
- AI Infrastructure: Performance and costs are key differentiators.
- Vertex (Enterprise AI): Customizable models tailored for enterprises.
- BigQuery (Data platform): Real-time analysis and decision-making.
- Cybersecurity: Enhanced by Mandiant since 2022.
- Applications: Including customer engagement or employee agents.
― Google: Little Engine That Cloud - App Economy Insights [Link]
What’s next:
- Browser based Agent - Project Jarvis: an AI technology that can autonomously take over a web browser to handle tasks like research and shopping.
- Waymo - closed massive funding round and has secured \(\$5.6\)B. mMajor backers are Andreessen Horowitz, Fidelity, and T. Rowe Price. Expansion would be driven by new funding through partnership with Uber.
- AI power - Alphabet is partnering with Kairos Tech to harness small nuclear reactors to power AI data centers.
- Search and competition: Google’s losing market share to TikTok and AI startups (Perplexity and OpenAI) but it is still the largest. Amazon’s search is catching up. TikTok and AI Chatbots are still tiny. Google’s decline in market share is likely primarily due to e-commerce based search on platform (Amazon).
Amazon: Still Day 1 For AI - App Economy Insights [Link]
On advertising: sponsored products remain a critical growth driver. Ad-supported Prime Video introduced in Q1 2024 automatically converted all Prime members to an ad-supported tier.
On lowering the cost to serve: 1) Expanding with over 15 new inbound buildings across the US, 2) Increasing same-day deliveries, 3) Advancing robotics and automation.
On pharmacy: significantly expanded with rapid delivery capability.
On Capex: aggressive infrastructure investments.
On Project Kuiper: Kuiper aims to provide fast, affordable internet via satellite. It is early in its journey but holds transformative potential for Amazon’s growth.
Tesla’s Cybercab could either compete with Uber’s platform or, as Khosrowshahi suggests, Cybercab fleet owners might choose to list their vehicles on Uber to maximize earnings. Uber’s reach and ability to cover diverse use cases—across vehicle sizes, geographies, and special needs—could lead to a hybrid model where Tesla AVs appear on Uber.
Tesla could ultimately leverage Uber’s scale and network, given the challenge of reaching a critical size in specific markets. AVs on Uber are already a reality with Waymo, and more will likely come.
― Tesla: Autonomy Gamble - App Economy Insights [Link]
Business Insights:
- Deliveries rebounded in Q3, leading to an auto gross margin improvement.
- Roughly 20% of Tesla‘s gross margin came from non-auto segments—nearly doubling from a year ago.
- Lower cost per vehicle, growth in non-auto segments, FSD revenue, growth in deliveries, and higher regulatory credit revenue contribute to operating margin.
- Free cash flow expanded and balance sheet remains stellar.
“We, Robot”s takeaways:
- Cybercab (robotaxi), Optimus (Humanoid Robot), Robovan
- FSD progress: promised to enable fully autonomous driving by 2026
- Market reaction: uncertain about the timeline
- Supercharger network: Most automakers have adopted Tesla’s North American Charging Standard (NACS).
- Market share: Tesla’s vehicles market share has stabilized in North America and Europe but noticeably improved in China.
- AI power: Musk still expects nearly 90,000 H100 clusters dedicated to training by the end of this year.
- Energy storage deployment
Comparing Tesla and Waymo:
- According to six SAE levels of driving automation (0 no automation, 1driver assistance, 2 partial automation, 3 conditional automation, 4 high automation, 5 full automation), Tesla’s FSD remains at level 2, while Waymo operates at level 4.
- Tesla relies on cameras and AI while Waymo relies on heavy hardware (LiDAR, radar, cameras).
- Waymo’s reliance on expensive hardware limits its ability to scale quickly (\(\$ 200\)K per vehicle). Tesla aims to scale faster by leveraging its existing fleet to train its AI models.
- Waymo has built trust with regulators by gradually deploying its vehicles, whileTesla faces regulatory hurdles particularly with the Cybercab.
Netflix: Crushing It Again - App Economy Insights [Link]
Deep Dive Into The Security for AI Ecosystem - Indiscrete Musings [Link]
_“*_This is an empirical law, not a fundamental physical law__. But the evidence is that it continues to scale. What we’re learning, however, is that it’s not enough, that we’ve now discovered two other ways to scale.*
One is post-training scaling. Of course, the first generation of post-training was reinforcement learning human feedback, but now we have reinforcement learning AI feedback, and all forms of synthetic data generated data that assists in post-training scaling.
And one of the biggest events and one of the most exciting developments is Strawberry, ChatGPT o1, OpenAI’s o1, which does inference time scaling, what is called test time scaling. The longer it thinks, the better and higher-quality answer it produces.”
― NVIDIA: The Age of AI - App Economic Insights [Link]
In an agent-first world, the traditional approach to A/B testing becomes obsolete. Instead of testing different button colors or copy variations for human users, companies like Amazon will need to optimize for agent interaction efficiency and task completion rates.
These A/B tests will target similar metrics as today: purchases, sign-ups, etc., employing LLMs to generate and test thousands of agent personas without the need for lengthy user testing cycles.
― Agent-Responsive Design: Rethinking the web for an agentic future - AI Tidbits [Link]
Several interesting vision for AI Agent world: 1) the death of traditional A/B testing, 2) switch from SEO to AEO (Agent Engine Optimization), 3) web moving from being bot blocked to bot embraced.
This is because AIs are inconsistent and weird, and often have different results across different models. For example, they are sensitive to small changes in spacing or formatting; they get more accurate when you tell them to “read the question again;” they seem to respond better to politeness (but don’t overdo it); and they may get lazier in December, perhaps because they have picked up on the concept of winter break.
― Getting started with AI: Good enough prompting - One Useful Thing [Link]
These ideas are important to learn, as they broaden the scope of what is possible with LLMs. For example, using these techniques, we can:
- Allow an LLM to access an external knowledge database.
- Enable complex, reasoning-based problems to be solved.
- Provide unlimited memory to an LLM by allowing the model to store and access prior information from a conversation.
― Advanced Prompt Engineering - Deep (Learning) Focus [Link]
Article covers CoT prompting, automatic prompting (interesting idea: “we could even consider our prompt as a group of trainable parameters that can be updated (e.g., using gradient descent or some other data-driven criteria) to generate a correct answer”), information retrieval, etc.
Energy Drink Economics - App Economy Insights [Link]
In my view, 2025 will be the year major AI agent frameworks compete for developers globally.
What makes these workflows special is their flexibility. The same principles we used for research papers can be applied to industry reports, technical documentation, or any complex text. The YouTube synthesis approach works just as well for conference talks, interviews, or training videos.
― How to use NotebookLM for personalized knowledge synthesis - AI Supremacy [Link]
New AI Agent based Applications:
- Google Learn About for education
- Perplexity as the advent of AI commerce, partnering with US campuses and Shopify.
- Amazon’s Multi Agent Orchestrator via AWS
- Google NotebookLM for researching, podcasting.
Google NotebookLM:
Capabilities
It stays focused on your sources - unlike ChatGPT, it shouldn’t hallucinate or bring in outside information
It can process multiple documents at once, finding connections between them
It generates natural-sounding podcast discussions about your content
It provides source citations for everything, linking directly to the original text
It’s completely free (for now)
Workflows (research papers and YouTube videos)
- Research papers:
- Overview phase: Create a discussion that focuses on the key methodology choices, main findings, limitations and gaps, and connections to existing research. Present it for a non-technical audience.
- Deep understanding: Ask about key assumptions in their methodology, explore alternative approaches they might have considered, and examine how their findings compare to related work.
- Synthesis phase: Compare and contrast these papers’ approaches and findings. Identify patterns, contradictions, and gaps that could inform future research.
- YouTube videos:
- Overview phase: Create a comprehensive discussion about AI agents, focusing on unique perspectives from each source.
- Research papers:
Tips and Pitfalls
- Don’t overload with too many documents at once
- Avoid overly broad instructions like “tell me everything important”
- Don’t skip the customization step
- Remember to specify your audience level (this drastically improves output quality)
We don’t want bias-free AI. We want an AI with biases that are explicit (we know exactly what it looks at), controllable ( we can influence how much it looks at a factor), and agreeable (the biases in the AI must be compatible with our standards of morality, ethics, and law).
― A look at Bias in Generative AI [Thoughts] - Artificial Intelligence Made Simple [Link]
The author pointed out sources of biases (process, dataset, model, and post-generation control mechanism). He highlighted that transparency is the solution. Technical transparency includes:
- Attention visualization tools
- Token-level confidence scores
- Explanation generation mechanisms
- Citation and source tracking
- Agentic architecture and separation of conerns
- Access to embedding models
And he also recommended several development practices to promote AI pipeline transparency: publishing open source models, creating synthetic data, creating transparent standards, and involving external auditors.
Why Data is an Incomplete Representation of Reality [Thoughts] - Artificial Intelligence Made Simple [Link]
This article argues that Data reflects our biases and values rather than providing an objective view of the world and data alone is insufficient for achieving superhuman AI. It offers three types of intelligence that are often overlooked in datasets: cultural intelligence, delusional intelligence, and subjective intelligence.
In my view, those are the gaps between AI and human. AI becomes human if those intelligence are acquired. However the question is, should AI become human first before becoming superhuman AI? It is true that human level is not skippable in the path to AGI?
Some interesting further discussion points implied by this blog:
How can we better incorporate cultural intelligence into AI training datasets and algorithms?
Other than broadening data or documenting practices, what’s more interesting is to develop AI system that can identify and adapt to different cultural contexts
What are the ethical implications of AI systems lacking delusional and subjective intelligence?
Be prone to perpetuating existing biases and discriminatory practices without subjective consideration. Limit problem solving capabilities (? But creativity of LLM can be tuned by setting parameters). Not able to adapt to cultural nuances.
What are the limitations of relying solely on quantitative metrics in evaluating AI performance?
Lead to exclusion of crucial qualitative factors; incentivize the optimization of narrow objectives rather than broader well-being; not able to capture the complex and nuanced nature of human intelligence.
Here are a few examples you’ve all experienced first-hand:
- Public Cloud enabled the SaaS economy
- The iPhone enabled the App economy
- Social media enabled the Creator economy
- LLMs gives rise to the Agentic economy
― Agentic Revolution - Startup Riders [Link]
How to use Perplexity in your daily workflow [Link]
Perplexity now has a desktop app. Fantastic application. Comparable or better than Google Search. For a learner like me, it’s a good tool to address my question efficiently and help with note taking.
Articles and Blogs
Enthusiasm for ChatGPT spread with Linton’s buy-in, prompting the company to launch a pilot program to identify key use cases. Today, ChatGPT is an integral part of Promega’s workflows, with over 1,400 custom GPTs used by 80% of the company.
Members of Promega’s Quality Assurance team automate customer requests and responses with a custom GPT that integrates with their Power Automate workflow. “With this AI-powered solution, we provide timely, accurate responses to over 250 quality surveys a year,” says Abigail David, Director of Quality Assurance. “The automation reduces internal workload by more than 600 hours annually and delivers key documents, like certifications and quality policies, effortlessly to our customers.”
- My Prospecting Pal GPT, which quickly identifies vital information about a given prospect and suggests potential Promega offerings. “The GPT can highlight key research initiatives that might benefit from Promega solutions, or even common interests between the salesperson and the prospect to enable a natural dialogue. This has cut our lead analysis time by 1–4 hours per prospect, allowing us to focus more on relationship building,” says Franchestia Flennory, a Promega Account Manager.
- Email Marketing Strategist GPT, which halves the time from content creation to campaign execution. In months, hundreds of marketing emails were deployed in half the usual time, saving 135 hours of work. “The time we get back from aligning on the strategy of emails can be invested into the user experience,” says Kari Siegenthaler, a Marketing Strategist with Promega. “I don’t know the last time I wrote an email without using this GPT.”
― Promega’s top-down adoption of ChatGPT accelerates manufacturing, sales, and marketing - OpenAI Blog [Link]
Rakuten’s goal is to become an “AI empowerment company.” They’re using Code Interpreter and RAG (retrieval-augmented generation) with OpenAI’s models to understand and extract value from complex, unstructured data, and the results have empowered customers and businesses in new ways:
- Previously, users had to wait days to get a response to a customer service ticket. “By using OpenAI’s API with RAG on our internal knowledge base, we’re now able to respond to and help users automatically,” Kaji said. This innovation has significantly improved response times and efficiency.
- Few people have time to wade through hundreds of user reviews when they’re shopping, so Rakuten is developing a feature that extracts key topics and summarizes reviews. “This will allow users to access and explore the information in a much more structured way,” Kaji said.
- Knowledge retrieval has also made a large impact on Rakuten’s B2B business. Rakuten consultants are now empowering merchants and enterprises with actionable insights from the company’s wealth of data, such as market analyses and sales trends.
― Rakuten pairs data with AI to unlock customer insights and value - OpenAI Blog [Link]
YouTube and Podcast
Gaming, Goats & General Intelligence with Frederic Besse - Google DeepMind [Link]
Google Research Engineering Team Lead discusses a future of very intelligent AI agents.
LangGraph Deep Dive: Build Better Agents - James Briggs [Link]
A tutorial of building an AI research agent using LangGraph.
Solving complex problems with OpenAI o1 models [Link]
This video demonstrates o1 models’ advanced reasoning across complex domains like programming.
Lecture Series in AI: “How Could Machines Reach Human-Level Intelligence?” by Yann LeCun - Columbia Engineering [Link]
Stanford CS229 I Machine Learning I Building Large Language Models (LLMs) [Link]
This Stanford lecture is about how to build LLMs, mainly focusing on practical training aspects, data handling, and evaluation methods.. It covers:
Pre-training phase
- Learn auto-regressive language modeling
- Understand tokenization (BPE method)
- Master cross-entropy loss calculation
- Track model progress through perplexity
Post-training phase (after ChatGPT era)
- Convert base models into AI assistants
- Apply evaluation benchmarks like MMLU
- Handle train-test contamination issues
Technical components
- Select proper model architecture
- Implement training algorithms
- Process training data
- Set up evaluation metrics
- Build system infrastructure
Dario Amodei: Anthropic CEO on Claude, AGI & the Future of AI & Humanity | Lex Fridman Podcast #452 [Link]
Papers and Reports
Large Language Models Are Human-Level Prompt Engineers [Link]
They introduced APE, a system for automatic prompt generation, which selects the most effective instructions for large language models (LLMs) to perform various tasks.
Automatic Prompt Optimization with “Gradient Descent” and Beam Search [Link]
They proposed an automatic prompt optimization method. Inspired by gradient descent, it generates textual “gradients” that identify prompt weaknesses and edits the prompt in the opposite semantic direction.
- Collecting errors made by the current prompt on the training data.
- Summarizing these errors via a natural language gradient.
- Using the gradient to generate several modified versions of the prompt.
- Selecting the best of the edited prompts.
- Repeating this process several times.
GrIPS: Gradient-free, Edit-based Instruction Search for Prompting Large Language Models [Link]
They introduced GRIPS (Gradient-free Instructional Prompt Search) as a gradient-free, edit-based method to improve natural language prompts for LLMs without needing gradient-based tuning.
RIPS takes human-designed instructions and automatically edits them to enhance performance. It involves random phrase-level edits like deletion, swapping, paraphrasing, and addition, which are scored based on task performance.
Large Language Models as Optimizers [Link]
This research introduces Optimization by Prompting (OPRO), an approach that leverages LLMs as optimizers by using natural language to describe optimization tasks. OPRO can be applied to linear regression, traveling salesman, and prompt optimization, where OPRO finds instructions that maximize task accuracy.
- Describing an optimization task in natural language.
- Showing an optimizer LLM examples of prior solutions to the optimization task along with their objective values.
- Asking the optimizer LLM to infer new / better solutions to the problem.
- Testing the inferred solutions via an evaluator LLM.
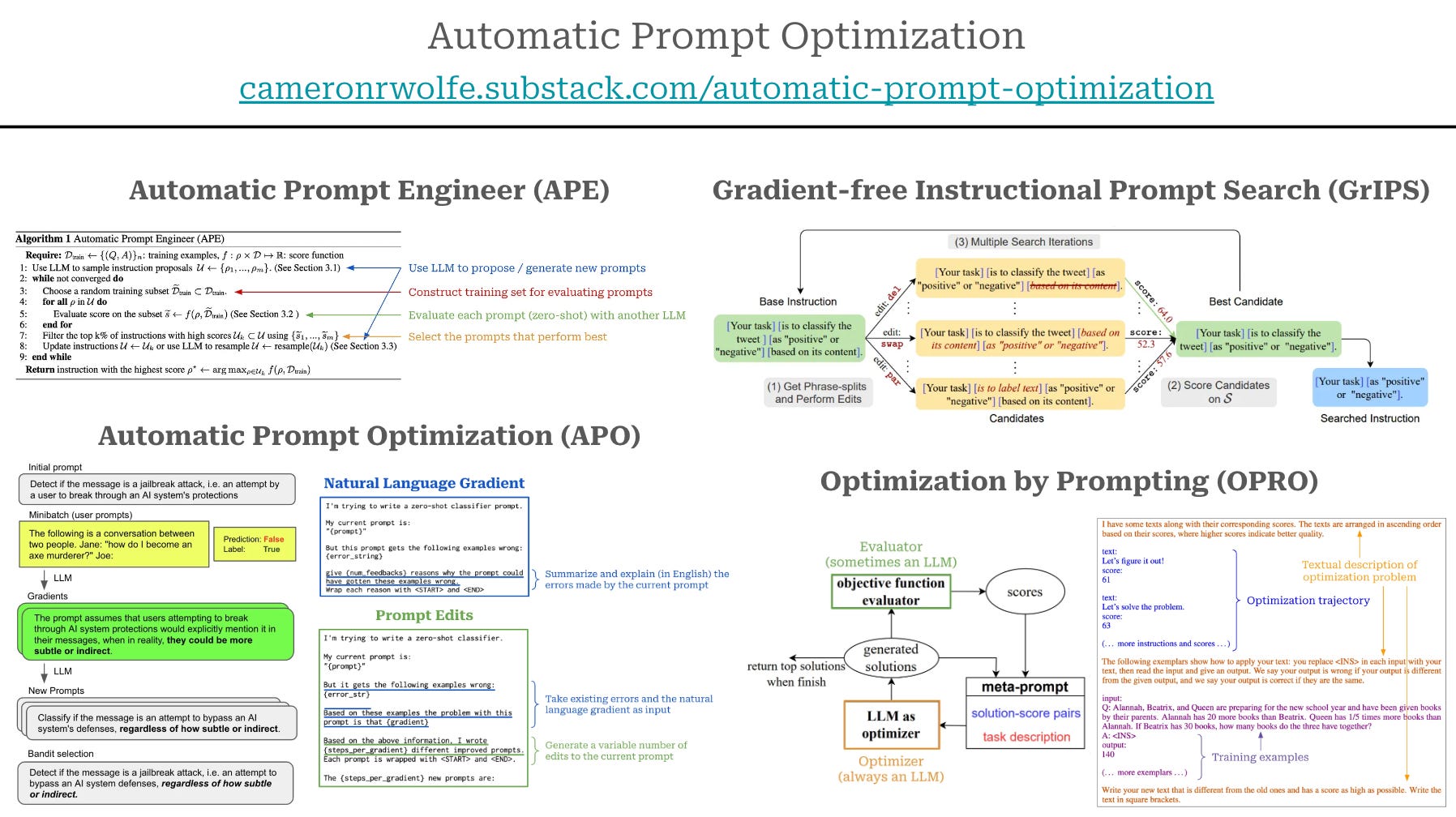
Prompting Guide 101 - Gemini for Google Workplace [Link]
GSM-Symbolic: Understanding the Limitations of Mathematical Reasoning in Large Language Models [Link]
Interesting findings on model variability: 1) Significant performance variations when questions are rephrased or when only numerical values are altered. 2) Models demonstrate robustness to superficial changes (e.g., proper names) but are highly sensitive to numerical changes.
Interesting findings on model complexity and fragility: 1) Model performance deteriorates as the number of clauses in a question increases, revealing challenges with handling complexity, 2) Adding irrelevant but seemingly relevant clauses leads to a performance drop of up to 65% in some models.
Insights in reasoning: 1) The decline in performance suggests LLMs rely on pattern matching rather than genuine logical reasoning, 2) Models replicate training data patterns rather than solving problems from first principles.
Thinking LLMs: General Instruction Following with Thought Generation [Link]
Current LLM has a problem of lacking internal reasoning processes before outputting responses. Explicit thinking can enhance performance on complex tasks, including creative writing and problem-solving, by allowing models to internally reason and plan responses. The author introduces Introduces Thought Preference Optimization (TPO) which allows LLMs to generate multiple thought-response pairs for each instruction, while a judge model evaluates responses, selecting the best and worst pairs for optimization.
Agent-as-a-Judge: Evaluate Agents with Agents [Link]
They introduced the Agent-as-a-Judge Framework to evaluate agentic systems, addressing limitations of existing evaluation methods like LLM-as-a-Judge by offering dynamic, step-by-step feedback throughout task-solving processes.
Difficulties handling numbers may stem from the fact that most models rely on autoregressive next token prediction pretext tasks during training, which might not be suitable for mathematical operations, or simply because a limited number of numerical reasoning tasks are included in the model’s training corpora. Nevertheless, it is known that performance can be improved using prompt techniques, indicating that relevant knowledge may already exist within LLMs.
Evaluating and enhancing probabilistic reasoning in language models - Google Research [Link]
What Are the Odds? Language Models Are Capable of Probabilistic Reasoning [Link]
This study introduces a benchmark dataset with question-answer pairs based on both idealized and real-world distributions. It enables systematic evaluation of LLMs’ probabilistic reasoning capabilities across three tasks: estimating percentiles, drawing samples, and calculating probabilities.
The technology has strikingly disparate effects across the productivity distribution: while the bottom third of scientists see little benefit, the output of top researchers nearly doubles.
Top scientists leverage their domain knowledge to prioritize promising AI suggestions, while others waste significant resources testing false positives.
82% of scientists report reduced satisfaction with their work due to decreased creativity and skill underutilization.
― Artificial Intelligence, Scientific Discovery, and Product Innovation [Link]
MIT PhD Aidan Toner-Rodgers’s working paper talking about some very interesting points. Indeed, AI has reshaped R&D process especially in natural science and material science where structured search is required .e.g drug discovery, climatology, etc. However, scientists with different degree of expertise (top and bottom scientists) achieve drastically different productivity with AI, giving bottom scientists less benefits. This characteristic has some consequences and implications
- Resources are misallocated to less promising AI suggestions. Human innovation and creativity is not encouraged and cultivated.
- Expertise is still required as AI only demonstrates its potential when complemented by human expertise. The judgment ability in leveraging AI’s potential is important.
- Skills have been shifted to prompting AI effectively. However, scientists feel an underutilization of expertise when working with AI.
A Survey on LLM-as-a-Judge [Link]
There are a lot of applications of LLM-as-a-Judge.
- Data annotation: labeling datasets with information such as sentiment, topic categorization, or relevance.
- Content critique: providing feedback on generated content such as articles, essays, or code.
- Domain-specific evaluations: evaluate the accuracy, completeness, and clarity of financial analyses or advice (in finance), and assess medical responses for correctness, compliance with guidelines, and patient safety (for medical Q&A).
Looking Inward: Language Models Can Learn About Themselves by Introspection [Link]
The researchers define introspection as “acquiring knowledge that is not contained in or derived from training data but instead originates from internal states”.
They conducted interesting experiments: finetuning LLMs to predict properties of their own behavior in hypothetical scenarios. It turns out that a LLM can predict itself better than other models predicting it, even those models are trained on the same data pool.
Conclusion is suprising - language models have knowledge about themselves that is neither contained in their training data nor inferable from it. The researchers developed a self-prediction training framework where models predict properties of their hypothetical responses.There is already LLM research areas in honesty, behaviors, etc. I believe this work is hugely contributing to these areas.
A Theoretical Understanding of Chain-of-Thought: Coherent Reasoning and Error-Aware Demonstration [Link]
Some interesting findings: 1) coherent CoT is better than traditional CoT because the former considers the connections between steps, 2) model is more sensitive to errors in intermediate reasoning steps than in the final answer
The authors proposed an error aware training method which works to incorporate both corretc and incorrect reasoning paths, enabling LLMs to recognize and handle potential reasoning errors.
Though businesses are doing their diligence on ROI and customization, they may miss crucial pieces of the implementation puzzle. Often, organizations discover too late that they’ve underestimated the importance of technical integration, ongoing support, and scalability. It’s a bit like buying a car based solely on fuel efficiency, only to realize later that service availability and ease of maintenance are just as critical over the long haul.
― 2024: The State of Generative AI in the Enterprise [Link]
Key Trends for 2024 onwards
There is a serious commitment from enterprise to AI integration in business strategies
The top use cases for generative AI focus on enhancing productivity and efficiency. These include:
- Code Copilots (51% adoption)
- Support Chatbots (31% adoption)
- Enterprise Search + Retrieval (28% adoption)
- Data Extraction + Transformation (27% adoption)
- Meeting Summarization (24% adoption)
There’s a growing trend towards autonomous AI agents capable of managing complex processes independently.
Businesses are focused on tools that deliver measurable value (ROI) and industry-specific customization, rather than simply looking for the cheapest option.
Industry-specific, verticalized AI applications are gaining momentum, particularly in:
- Healthcare ($500 million in enterprise spending)
- Legal ($350 million in enterprise spending)
- Financial Services ($100 million in enterprise spending)
- Media and Entertainment ($100 million in enterprise spending)
Companies prefer multi-model strategies. This has led to a decline in OpenAI’s dominance, while Anthropic is gaining market share.
Retrieval-augmented generation (RAG) has become the dominant design pattern, with 51% adoption. Meanwhile, agentic architectures are emerging, now powering 12% of implementations.
There is a talent drought as AI engineering becoming more sophisticated.
There’s a growing trend towards companies building their own AI solutions in-house.
Previously, in 2023, a large majority of enterprises (80%) relied on third-party vendors for their generative AI software
In 2024, the split between building and buying is almost even, with 47% of solutions developed internally and 53% sourced from vendors
This shift suggests a growing confidence among enterprises in their ability to develop and implement their own AI tools.
while there’s a trend towards building in-house solutions, companies are not abandoning vendors entirely. The sources still highlight the importance of vendors, especially for companies lacking the resources or expertise for in-house development. The even split between building and buying suggests a hybrid approach is emerging, where companies strategically choose which solutions to develop internally and which to procure from vendors.
Articles and Blogs
Magentic-One: A Generalist Multi-Agent System for Solving Complex Tasks - Microsoft Research [Link]
Magentic-One is built on Microsoft’s AutoGen framework. It employs a unique dual-loop architecture where the Orchestrator manages both task and progress ledgers. This is an early movement of building generalist agentic systems. Other current LLM-based applications like RAG will also benefit from this type of system.
Introducing Internal Knowledge Search and Spaces - Perplexity [Link]
Internal Knowledge Search and Spaces enable simultaneous searches of organizational files and the web. This feature addresses the need for a unified tool to access both internal and external data, leveraging advanced LLMs like GPT-4 and Claude 3 to enhance search efficiency and relevance.
After the introduction of ChatGPT, there was a 21% decrease in the weekly number of posts in automation-prone jobs compared to manual-intensive jobs. Writing jobs were affected the most (30.37% decrease), followed by software, app, and web development (20.62%) and engineering (10.42%).
To stay competitive, employees must engage in continuous learning and upskilling. In their book Prediction Machines, authors Ajay Agrawal, Joshua Gans, Avi Goldfarb argue that AI is shifting the focus of work away from predictive tasks to those requiring human judgment and decision-making.
― Research: How Gen AI Is Already Impacting the Labor Market - Harvard Business Review [Link]
Research reveals impact of GenAI applications (ChatGPT and image-generating AI) in jobs (manual intensive jobs such as data and office management, video services, and audio services; automation prone jobs such as writing, software, app, web dev, and engineering, and image-generating jobs such as graphic design and 3D modeling ) to see challenges and opportunities in shifting markets.
They found that Gen AI “led to nearly immediate decreases in posts for online gig workers across job types, but particularly for automation-prone jobs. “ It shows a growing trend of job replacement.
Suggestions are continuous learning, enhancing human judgment and decision making, to be able to ask right questions, prompt efficiently, and avoid blindly taking responses.
How Much GPU Memory is Needed to Serve a Large Language Model (LLM)? [Link]
Addresing a common LLM interview question “How much GPU memory is needed to serve a Large Language Model (LLM)?”.
\[ M = ({P \times 4B \over {32/Q}}) \times 1.2 \] where P is model size, 4B is 4 bytes used per paramter, Q is the number of bits for loading the model (16 bit or 32 bit). 1.2 accounts for a 20% overhead.
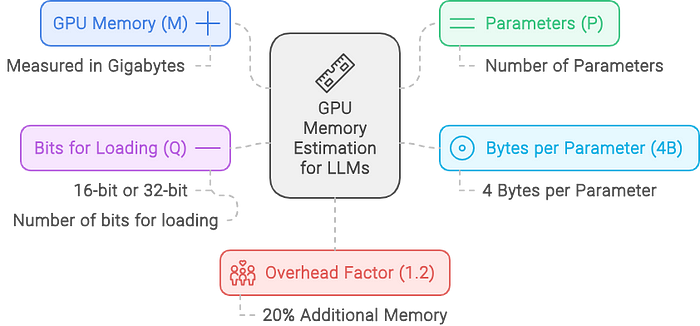
AI Agent Stack [Link]
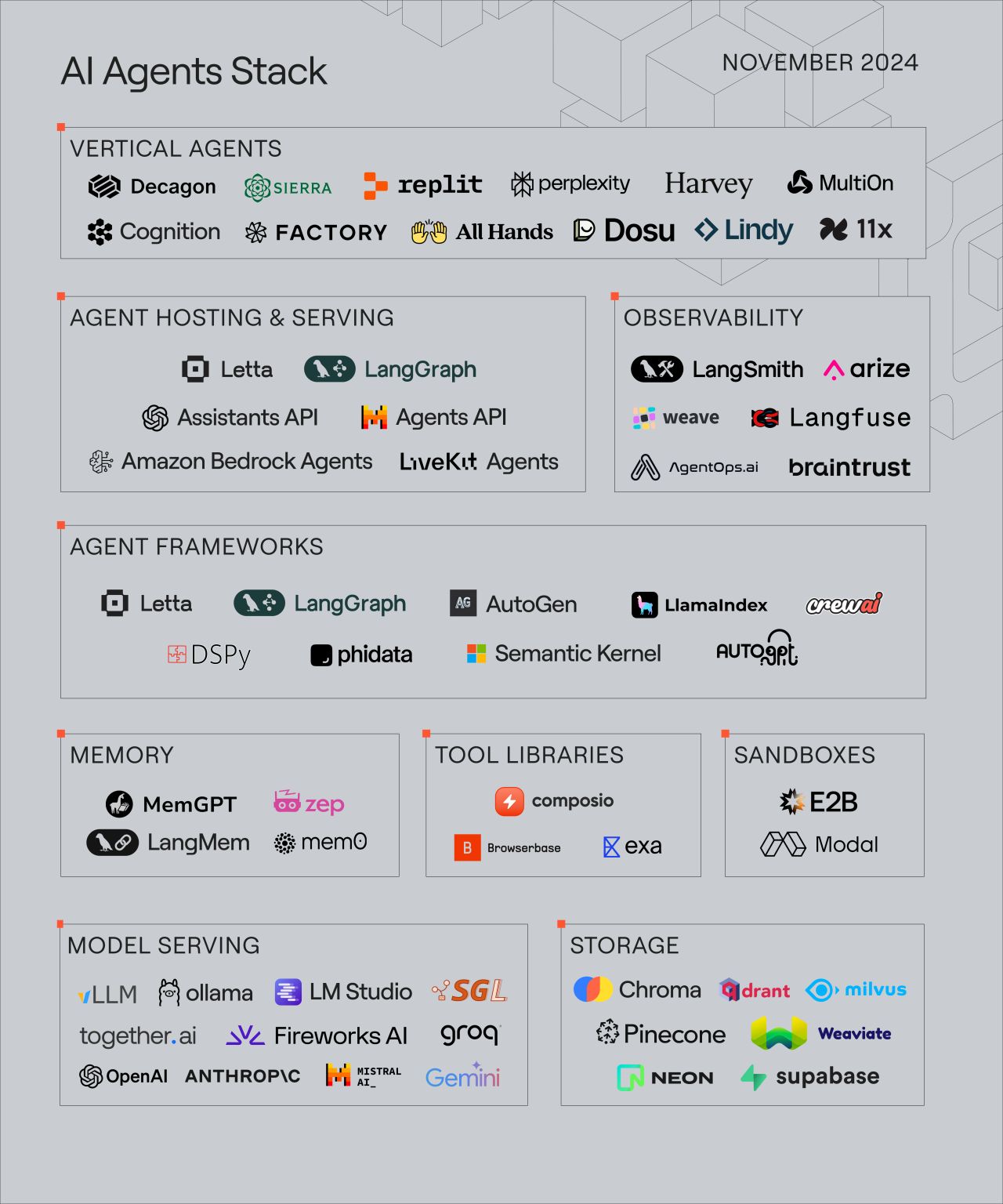
GitHub
OpenCoder: The Open Cookbook for Top-Tier Code Large Language Models [Link]
News
Introducing ChatGPT search - OpenAI [Link]
Perplexity introduces AI-powered finance tool, offering real-time stock analysis and historical data for developers. [Link]
VS Code now supports GitHub Copilot chat search and visualization [Link]
Cognitive Scientist Gary Marcus Says AI Must Be Regulated. He Has a Plan [Link]
Among several points made by the article, two were caught by my eyes:
Elon Musk presents a complex figure in the AI landscape, as one of the first to issue warnings about potential risks of AI, and also actively involved in developing AI through his company. This duality raises questions about his stance on AI and how he reconciles his concerns with his entrepreneurial pursuits.
Marcus proposes a shift from the current “System One” thinking in AI, which is fast and reflexive but prone to errors, to a “System Two” approach that emphasizes deliberate reasoning and abstraction.