2025 January - What I Have Read
Substack
How Meta Plans To Crank the Dopamine Machine With Infinite AI-Generated Content - The Algorithmic Bridge [Link]
This article discussed AI’s most dangerous potential - its ability to manipulate and addict humans through hyper-targeted entertainment. This trend, spearheaded by companies like Meta, risks reshaping human cognition and agency, raising existential questions about freedom, pleasure, and the future of society.
One good point is that the killer robots are brain-hacking entertainment. A very plausible dystopia involves technology (e.g., AI-driven entertainment) manipulating human attention and cognition for profit. Traditional TV was a prototype of mental manipulation but lacked personalization. Current platforms such as Netflix and TikTok use algorithms to cater to preferences but still feel limited. Future AI will create hyper-personalized content tailored to individual preferences in real-time, exploiting human psychology. Meta’s generative AI plans are the next step toward addictive, manipulative entertainment. Meta announced that AI content creators will be designed to enhance engagement on platforms like Facebook and Instagram. Connor Hayes, Meta’s VP for generative AI, explained how AI accounts will create and share engaging content.
The Five Stages of AGI Grief - Marcus on AI [Link]
Marcus uses the framework of the Kübler-Ross model of grief to describe the emotional responses people are having (or will likely have) to the ongoing developments in Artificial General Intelligence (AGI). He argues that many people are not yet facing the reality of AGI and are likely to go through similar stages of grief as it gets closer.
- Denial: Many people, including some experts, are still in denial about the possibility and speed of AGI development. They dismiss the progress, underestimate its potential, or claim it's decades away.
- Anger: Once denial fades, anger emerges, often directed at those perceived as enabling or hyping AGI. This can be targeted at AI researchers, tech companies, or even the technology itself.
- Bargaining: In this stage, people try to find ways to control or mitigate AGI, often through unrealistic expectations or proposed solutions.
- Depression: As bargaining fails, a sense of profound unease and hopelessness may set in. This is the realization that AGI could fundamentally change society in ways that are difficult to predict or control, leading to feelings of powerlessness.
- Acceptance: This is the final stage, where people begin to accept the reality of AGI and its potential impact. This isn't necessarily cheerful, but it's characterized by a shift from denial and fear towards a more realistic view.
The Taiwan posts - Noahpinion [Link]
Disney Paid Off Trump for a Reason - BIG by Matt Stoller [Link]
Fubo, a sports streaming service, had previously won a preliminary injunction against a joint venture between Disney, Fox, and Warner Bros, arguing that the venture was an illegal merger. However, Fubo's stock wasn't performing well, leading Fubo CEO David Gandler to sell a controlling stake in his company to Disney.
Here are the rationales behind this decision, according to the sources:
- Fubo's CEO, David Gandler, profited from winning an antitrust suit and joined forces with a large corporation. Instead of being an underdog fighting against major corporations, Fubo has now joined forces with one of them. Fubo will now have Disney's resources, while its leaders imagine that it will operate somewhat independently.
- Disney made a $16 million payment for defamation against Trump, which is considered questionable by legal analysts, in order to gain credibility with Trump. The aim of this was to ensure that government enforcers would not interfere with the deal.
- Fubo's leaders may be ignoring the risks involved in the merger. They are potentially exhibiting a kind of "malevolent naivete" and airbrushing away their own violation of the law.
The sources suggest that Fubo's leadership may not be considering some of the risks associated with mergers. Mergers carry significant risk, and they can fall apart for a variety of reasons. During the 18-24 months that it takes to clear financing and regulatory hurdles, a company under contract to be sold cannot make significant strategic decisions or investments, while the purchaser can do whatever they want. If the deal falls apart, the company that was to be sold could be in a significantly worse position.
The sources point out that there is a possibility that another private litigant could take Fubo's place and sue, using the legal precedent set by Fubo. This is evidenced by a letter sent by EchoStar to the court, in which the company states that it's considering suing along the same lines as Fubo. This may not matter to Disney, since they now control Fubo, but it should be a source of concern for Fubo's leadership team who have essentially bet their company on a violation of the law.
A private litigant, such as EchoStar, could take Fubo's place and sue Disney, Fox, and Warner Bros, using the same legal arguments that Fubo successfully used to win a preliminary injunction. This is a possibility because the legal precedent set by Fubo remains, even though Fubo is now under Disney's control.
Here's why this could be problematic for Fubo but not necessarily for Disney:
- Fubo is in a vulnerable position due to the merger agreement. While the deal is pending, Fubo is restricted in its strategic decision-making and investments, effectively putting the company in "limbo". This means Fubo cannot make significant moves to respond to a new lawsuit.
- Disney, as the purchaser, is not similarly restricted. They can continue to operate as they see fit. They have the resources to handle a new legal challenge.
- If the merger fails, Fubo will have wasted 18-24 months with the potential for no significant strategic moves. It could end up in a weakened state compared to competitors who were not in a merger process. The company might even become "a limping and probably dead company". Failed mergers can also lead to leadership changes, such as the CEO getting fired.
- Disney has already taken steps to ensure the deal's success, including a payment to gain credibility with the current administration. While another lawsuit could present a challenge, Disney has the resources and political connections to navigate it, which Fubo does not.
- The incentive to complete the deal is different for Disney and Fubo. Disney will remain a major player regardless of the deal's outcome. However, Fubo's future is heavily dependent on the merger. This makes Fubo more vulnerable if the deal is challenged.
The rise and fall of "fact-checking" - Silver Bulletin [Link]
The main opinion of this article is that Meta's decision to replace fact-checkers with a community notes system is justifiable because fact-checkers have been politically biased and have not effectively addressed the issue of misinformation.
While the author agrees with Zuckerberg's decision, they also acknowledge that Zuckerberg's motivations may not be high-minded, but rather driven by political pressure and business incentives. Despite that, the author thinks the move is "pointing in the right direction," and agrees with Zuckerberg's claim that fact-checkers have been too politically biased. The author also admits their own biases and that Community Notes is a new program that might also have problems.
US Banks: Profits Surge - App Economy Insights [Link]
CES 2025: AI Takes Over - App Economy Insights [Link]
a16z's big ideas in tech for 2025 - ben lang's notes [Link]
Andreessen Horowitz’s list of big ideas in tech for 2025:
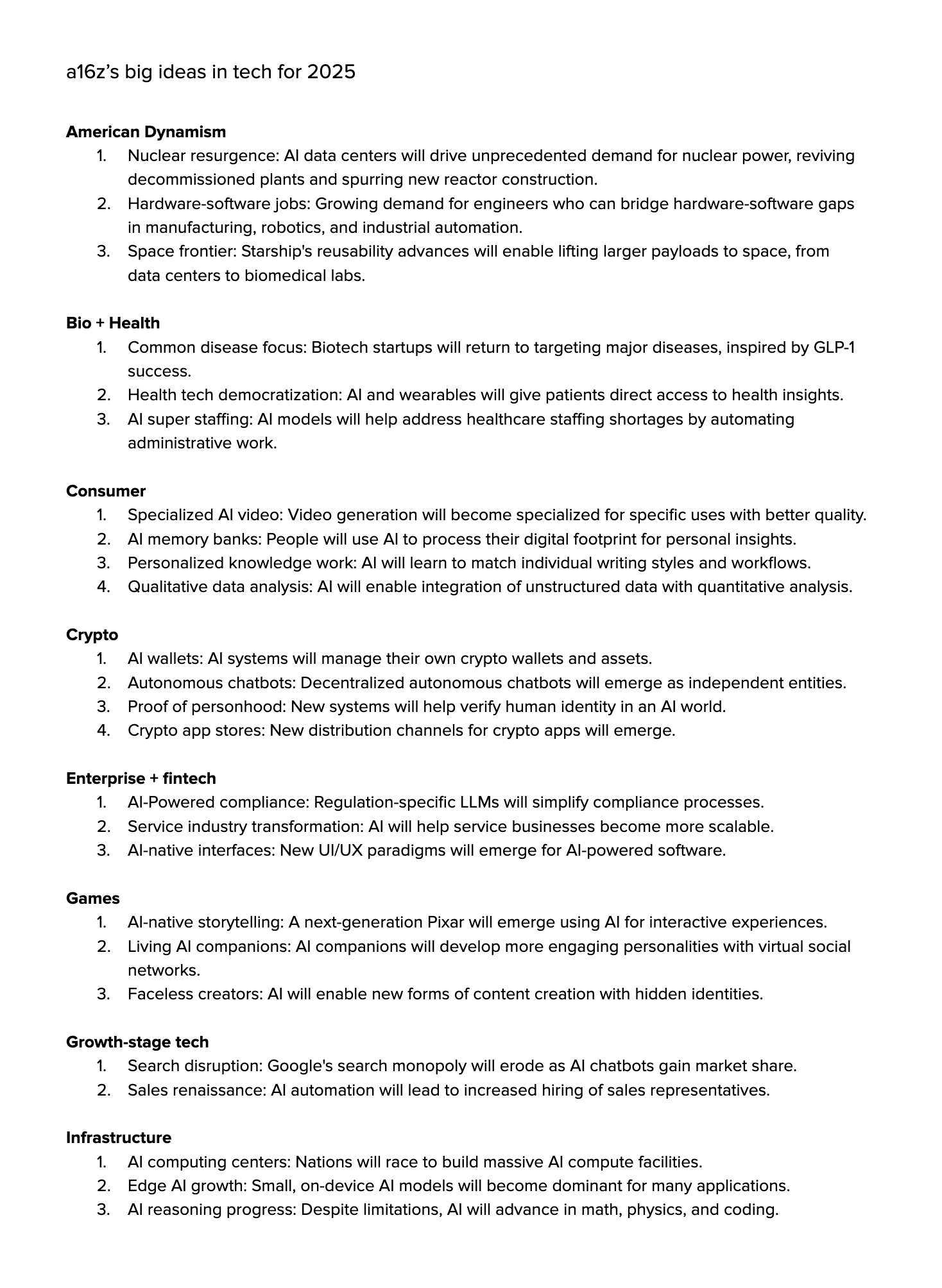
How AI-assisted coding will change software engineering: hard truths - The Pragmatic Engineer [Link]
Great article!
This "70% problem" suggests that current AI coding tools are best viewed as:
- Prototyping accelerators for experienced developers
- Learning aids for those committed to understanding development
- MVP generators for validating ideas quickly
Current tools mostly wait for our commands. But look at newer features like Anthropic's computer use in Claude, or Cline's ability to automatically launch browsers and run tests. These aren't just glorified autocomplete - they're actually understanding tasks and taking initiative to solve problems.
Think about debugging: Instead of just suggesting fixes, these agents can:
- Proactively identify potential issues
- Launch and run test suites
- Inspect UI elements and capture screenshots
- Propose and implement fixes
- Validate the solutions work (this could be a big deal)
― The 70% problem: Hard truths about AI-assisted coding - Elevate [Link]
Great pragmatic article! And it's well-said in the end: "Software quality was (perhaps) never primarily limited by coding speed...The goal isn't to write more code faster. It's to build better software. "
AI tools help experienced developers more than beginners. This is similar to the fact that AI helps top biologists to be successful more than normal biologists. The results and efficiency of AI usage differ based on users' domain expertise. This is called 'knowledge paradox'. AI can help to get the first 70% job done quickly, but the efforts on the final 30% have diminishing returns. This is called 'AI learning curve paradox'.
o1 isn’t a chat model (and that’s the point) - Latent Space [Link]
Provide Extensive Context: Give 10x more context than you think is necessary. This includes details about previous attempts, database schemas, and company-specific information. Think of o1 like a new hire that needs all the relevant information to understand the task. Put the context at the end of your prompt.
Use tools like voice memos to capture context and paste transcripts. You can also save reusable segments of context for future use. AI assistants within other products can help extract context.
Focus on the Desired Output: Instead of telling o1 how to answer, clearly describe what you want the output to be. Let o1 plan and resolve its own steps, leveraging its autonomous reasoning.
Define Clear Evaluation Criteria: Develop specific criteria for what constitutes a "good" output so that o1 can evaluate its own output and improve. This moves the LLM-as-Judge into the prompt itself. Ask for one specific output per prompt.
Be Explicit About Output Format: o1 often defaults to a report-style output with numbered headings. Be clear if you need complete files or other specific formats.
Manage Context and Expect Latency: Since o1 is not a chat model, it will not respond in real time, like email. Make sure you can manage and see the context you are providing to the model. o1 is better suited to high-latency, long-running tasks.
The Deep Roots of DeepSeek: How It All Began - Recode China AI [Link]
Liang's Visions from his first public interview in May 2023:
AI Development:
- Liang aims to build AGI, not just improve existing models like ChatGPT.
- He prioritizes deep research over quick applications, requiring more resources.
- He sees AI as a way to test ideas about human intelligence, like whether language is key to thought.
- He plans to share DeepSeek’s results publicly to keep AI accessible and affordable.
Company Culture & Innovation:
- He hires based on ability, creativity, and passion, preferring fresh graduates for key roles.
- Employees should have freedom to explore and learn from mistakes.
- It can't be forced or taught.
- A shared pace and curiosity drive the team, not strict rules or KPIs.
Competition:
- Startups can still challenge big companies since AI tech is evolving.
- No one has a clear lead in AI yet.
- LLM applications will become easier, creating startup opportunities for decades.
- AI believers stay in for the long run.
- Unconventional approaches can be a game-changer.
Resources & Funding:
- Securing GPUs and a strong engineering team is crucial.
- Traditional VC funding may not fit DeepSeek’s research-heavy approach.
- Innovation is costly, and some waste is inevitable.
- GPUs are a solid investment as they hold value.
Is DeepSeek the new DeepMind? - AI Supremacy [Link]
Implications for the AI Industry:
- DeepSeek's emergence challenges the dominance of Western AI firms like Google DeepMind, Meta, and OpenAI. The success of DeepSeek suggests that open-source models can outperform proprietary ones. It also calls into question the massive spending on AI infrastructure by Big Tech companies.
- Its cost-effectiveness is causing enterprises to rethink their AI strategies. The availability of high-performing, cheaper models could disrupt the business model of companies that rely on expensive, proprietary models.
- Its achievements indicate that China is becoming a leader in AI, particularly in inference-time compute and compute efficiency. This development raises concerns about America's shrinking lead in artificial intelligence.
- Its open-source approach is seen as essential to keeping AI inclusive and accessible. The ability to run powerful models on a laptop could decentralize AI development and reduce reliance on Big Tech.
Arguments about US vs. China in AI:
- The article suggests that the U.S. is losing its lead in AI innovation due to its focus on "Tycoon capitalism" and protectionist policies. The U.S. government's export controls on semiconductors, while intended to slow China's progress, may be inadvertently fueling China's self-reliance and innovation.
- China has advantages in areas such as manufacturing, go-to-market strategies, talent (STEM programs and ML researchers), and patents. China's progress in various overlapping industries creates a "mutually reinforcing feedback loop". The article implies that Chinese work culture of empowering workers with autonomy and collaboration is a strong contrast to the grueling work schedules, rigid hierarchies, and internal competition that are common in Chinese tech firms.
- The article criticizes the massive AI infrastructure projects in the U.S. (dubbed "Project Oracle") as a scheme by the financial elite to control the future of AI. The author argues that these projects prioritize the interests of Big Tech and the financial elite over those of regular citizens and that these AI infrastructure projects are primarily intended to redistribute wealth globally to the elite.
Concerns about AI's Impact:
- The author acknowledges concerns that AI could lead to wage deflation, particularly in white-collar jobs where AI can automate tasks.
- It questions the assumption that AI will create more jobs than it displaces, noting that AI coding tools could negatively impact software engineers.
- It also raises concerns about the potential for misuse of AI, including the use of AI for "authoritarian" control and as a weapon in trade wars. There are also concerns about the potential for backdoors, Trojans, model inversion attacks, sensitive information inference, and automated social engineering via the release of attractive but cheap AI services.
Additional Info:
- DeepSeek is an offshoot of a quantitative hedge fund, High-Flyer, and is fully funded by them.
- It is noted for being more transparent about its methods compared to some Western AI firms.
- Its mission is to "unravel the mystery of Artificial General Intelligence (AGI) with curiosity". They focus on open-source development, research-driven innovation, and making advanced AI accessible to all.
Monopoly Round-Up: China Embarrasses U.S. Big Tech - BIG by Matt Stoller [Link]
- DeepSeek, a Chinese AI firm, developed cost-effective AI models that rival U.S. models and released them on an open-source basis. This is a significant accomplishment, especially since the U.S. has placed export controls that prevent China from accessing the best chips. DeepSeek's approach focused on efficiency, rather than raw computing power, which challenges the assumption that computing power is the primary competitive barrier in AI. This development is considered embarrassing and threatening to big tech and U.S. security.
- The U.S. has heavily invested in AI, with tech giants spending billions on data centers and infrastructure, betting that these investments will provide a competitive advantage. However, DeepSeek’s success suggests that this approach may be flawed. The sources suggest that the U.S. strategy of denying top chips to China may also be ineffective.
- The sources argue that betting on monopolistic national champions is a disastrous national security strategy. It points out that history shows that monopolies are slow to innovate. The U.S. needs to prioritize competition over protecting monopolies. The sources criticize large U.S. tech firms (Meta, Microsoft, Google, Amazon, Apple) for becoming slothful bureaucracies that are not very good at developing and deploying technology.
- Chinese policy is noted to be more aggressive in forcing competition in some sectors. China's electric vehicle industry is cited as an example of this. The Chinese government's crackdown on its big tech firms and financial sector is also mentioned as a move that has seemingly benefited the economy by driving innovation. The success of companies like ByteDance and DeepSeek is mentioned as evidence of this.
- The sources highlight that U.S. anti-monopoly laws take too long to take effect. It uses the example of the Federal Trade Commission's case against Facebook for its acquisition of Instagram and WhatsApp. This case highlights how companies like Facebook acquire and bury innovative competitors rather than compete. It argues that if Facebook had been broken up, there would be tremendous innovation in social networking.
- The sources express uncertainty about the future of AI, noting it might not live up to expectations. It also notes that the competitive advantages in AI are not as straightforward as previously thought.
In a rare interview for AnYong Waves, a Chinese media outlet, DeepSeek CEO Liang Wenfeng emphasized innovation as the cornerstone of his ambitious vision:
. . . we believe the most important thing now is to participate in the global innovation wave. For many years, Chinese companies are used to others doing technological innovation, while we focused on application monetization—but this isn’t inevitable. In this wave, our starting point is not to take advantage of the opportunity to make a quick profit, but rather to reach the technical frontier and drive the development of the entire ecosystem.
― 7 Implications of DeepSeek’s Victory Over American AI Companies - The Algorithmic Bridge [Link]
"Every job is a bundle of tasks.
Every new technology wave (including the ongoing rise of Gen AI) attacks this bundle.
New technology may substitute a specific task (Automation) or it may complement a specific task (Augmentation)"
Extend this analogy far enough, and you get this:
Once technology has substituted all tasks in a job bundle, it can effectively displace the job itself.
Of course, there are limits to this logic. This can only be true for a small number of jobs, which involve task execution only.
But most jobs require a lot more than mere task execution.
They require ‘getting things done’. They require achievement of objectives, accomplishment of outcomes.
In other words, most jobs involve goal-seeking.
This is precisely why previous generations of technologies haven’t fully substituted most jobs. They chip away at tasks in the job bundle without really substituting the job entirely.
Because humans retain their right to play because of their ability to plan and sequence tasks together to achieve goals.
In most previous instances, technology augments humans far more than automating an entire job away.
And that is because humans possess a unique advantage: goal-seeking.
― Slow-burn AI: When augmentation, not automation, is the real threat - Platforms, AI, and the Economics of BigTech [Link]
AI agents are the first instance of technology directly attacking and substituting goals within a role or a team.
In doing so, they directly impact power dynamics within an organization, empowering some roles and weakening others, empowering some teams and weakening others.
― How AI agents rewire the organization - Platforms, AI, and the Economics of BigTech [Link]
This is a brilliant article.
Goal-seeking, for the first time, can be performed by technology.
- Scope of the role: Effectively, a goal-seeking AI agent can unbundle a goal from the role. They reduce the scope of the role.
- Scope of the team: They displace the role entirely in a team if the team can now achieve the same goal using an AI agent.
- Rebundling of roles: Role B is eliminated not because its tasks were fully substituted by technology, nor because its goals were fully substituted by technology, but because the scope of the role no longer justified a separate role.
- Reworking power structures: Teams have voting rights on the relevance of Roles. The fewer teams speaking to a role’s contributions, the lower the negotiating power for that role within the organization.
- Roles unbundle, teams rebundle: this cycle of unbundling and rebundling across roles and teams is inherent to the organization of work. AI isn’t fundamentally changing goal-seeking and resource allocation. It is merely inserting itself into the organization and re-organization of work.
YouTube and Podcasts
2025 Predictions with bestie Gavin Baker - All-In Podcasts [Link]
Interesting discussions about new year predictions. Here is a summary of the predictions:
Chamath Palihapitiya:
- Biggest Political Winner: Fiscal conservatives. He believes austerity will reveal waste and fraud in the US government and that this will spill over to state elections.
- Biggest Political Loser: Progressivism. He predicts a repudiation of class-based identity politics in multiple Western countries.
- Biggest Business Winner: Dollar-denominated stablecoins, which he believes will grow substantially and challenge the dominance of Visa and Mastercard.
- Biggest Business Loser: The "MAG 7" companies will see a drawdown in absolute dollars due to high concentration in the indices. He suggests that these companies may not be able to maintain their high valuations, though they are good businesses.
- Biggest Business Deal: The collapse of traditional auto OEMs and a wave of auto mega-mergers, triggered by Tesla's strong position.
- Most Contrarian Belief: A banking crisis in a major mainline bank, triggered by the total indebtedness of Pax America and the impact of higher interest rates.
- Best Performing Asset: Credit Default Swaps (CDS) as an insurance policy against a potential default event.
- Worst Performing Asset: The software industrial complex, or large, bloated enterprise software companies.
- Most Anticipated Trend: Small, arcane regulatory changes related to the supplemental loss ratio that allow the US to kick the debt can down the road.
- Most Anticipated Media: The enormity of files that will be declassified and released by the Trump administration.
- Prediction Market: The MAG 7 representation in the S&P 500 shrinks below 30%.
David Friedberg:
- Biggest Political Winner: Young political candidates, marking a trend of a shift towards younger leaders.
- Biggest Political Loser: Pro-war neoconservatives. He believes they will lose out to figures like JD Vance and Elon Musk.
- Biggest Business Winner: Autonomous hardware and robotics, citing the rise of humanoid robots and their applications.
- Biggest Business Loser: Old defense and aerospace providers, like Boeing and Lockheed Martin. He predicts a shift towards more tech-oriented and rationalized spending in defense. He also thinks Vertical SaaS companies will struggle as AI replaces their services.
- Biggest Business Deal: Massive funding deals for hardware-based manufacturing buildout in the United States, potentially involving government support.
- Most Contrarian Belief: A dramatic rise in socialist movements in the United States, fueled by economic inequality and disruption from AI.
- Best Performing Asset: Chinese tech stocks or ETFs, based on potential deals between the US and China and the strong fundamentals of Chinese tech companies.
- Worst Performing Asset: Vertical SaaS companies again as AI replaces the practices. Also legacy car companies and real estate because of overbuilding and high debt.
- Most Anticipated Trend: The announcement of buildout of nuclear power in the United States.
- Most Anticipated Media: AI Video Games with dynamic story lines
- Prediction Market: Microsoft, AWS, and Google Cloud Revenue Growth.
Gavin Baker:
- Biggest Political Winner: Trump and centrism; also Gen X and Elder Millennials.
- Biggest Political Loser: Putin, due to Europe rearming, which shifts US resources to the Pacific, and Trump's likely tougher stance.
- Biggest Business Winner: Big businesses that use AI thoughtfully, and the robotics industry, as well as companies that make high bandwidth memory.
- Biggest Business Loser: Government service providers with over 35% of their revenue coming from the US government. He also thinks Enterprise application software will be hurt by AI agents
- Biggest Business Deal: A wave of M&A after a period of inactivity and something significant happening with Intel. Also, he thinks independent AI labs will get acquired.
- Most Contrarian Belief: The US will experience at least one year of greater than 5% real GDP growth due to AI and deregulation. He also thinks frontier AI labs will stop releasing their leading-edge models.
- Best Performing Asset: Companies that make high bandwidth memory (HBM).
- Worst Performing Asset: Enterprise application software.
- Most Anticipated Trend: AI will make more progress per quarter in 2025 than it did per year in 2023 and 2024, due to scaling performance through reasoning, pre-training, and test time compute.
- Most Anticipated Media: Season 2 of 1923
- Prediction Market: US Treasury Market Report on Federal Debt in December 2025 above or below $38 trillion
- UFOs: Believes there is a 25% chance the US government is sitting on knowledge of extraterrestrial life.
Jason Calacanis:
- Biggest Business Winner: Tesla and Google for AI and Robotics
- Biggest Business Loser: Open AI
- Biggest Business Deal: Partnerships between Amazon, Uber, Tesla, and Waymo for autonomy, delivery, and e-commerce
- Most Contrarian Belief: Open AI will lose its lead and its nonprofit-to-for-profit transition and become the number four player in AI.
- Best Performing Asset: MAG 7 stocks
- Worst Performing Asset: Legacy car companies and Real Estate.
- Most Anticipated Trend: Exits and DPI will shower down, along with a surge in M&A and IPOs
- Most Anticipated Media: Legacy media outlets owned by billionaires attempting to steer towards the middle
- Prediction Market: Over or under 750,000 deportations by Trump in the first year of office
Building Anthropic | A conversation with our co-founders - Anthropic [Link]
WTF is Artificial Intelligence Really? | Yann LeCun x Nikhil Kamath | People by WTF Ep #4 - Nikhil Kamath [Link]
The Next Frontier: Sam Altman on the Future of A.I. and Society - New York Times Events [Link]
LA's Wildfire Disaster, Zuck Flips on Free Speech, Why Trump Wants Greenland [Link]
Text, camera, action! Frontiers in controllable video generation - William (Bill) Peebles [Link]
Best of 2024 in Agents (from #1 on SWE-Bench Full, Prof. Graham Neubig of OpenHands/AllHands) - Latent Space [Link]
The State of AI Startups in 2024 [LS Live @ NeurIPS] - Latent Space [Link]
Best of 2024 in Vision [LS Live @ NeurIPS] - Latent Space [Link]
Red-pilled Billionaires, LA Fire Update, Newsom's Price Caps, TikTok Ban, Jobless MBAs - All-In Podcast [Link]
NVIDIA CEO Jensen Huang Keynote at CES 2025 - NVIDIA [Link]
CES 2025 is the world's biggest tech expo. Each January, CES kicks off the tech year by highlighting everything from groundbreaking gadgets to the processors driving our digital world.
NVIDIA's CES announcements showcased its dominance in the AI chip market while highlighting its bold expansion into emerging, high-growth sectors. By emphasizing robotics, autonomous vehicles, and broader accessibility to AI, NVIDIA demonstrated its commitment to staying central to this wave of innovation.
Highlights:
GeForce RTX 50 Series GPUs
NVIDIA unveiled its latest GeForce RTX 50 series GPUs, powered by the advanced Blackwell architecture and set to launch in January. These GPUs deliver significant improvements in gaming and AI performance, with the flagship RTX 5090 priced at \(\$1,999\) and the RTX 5070 at \(\$549\), surpassing the RTX 4090, which debuted at \(\$1,599\) in 2022.
The 50 series also introduces DLSS 4, a cutting-edge Deep Learning Super Sampling technology that employs a transformer-based architecture to generate three AI-rendered frames for every traditionally rendered one, enhancing graphics quality and gaming experiences. NVIDIA partnered with Micron to supply memory chips for these GPUs.
Although GeForce RTX GPUs contributed only 9% of NVIDIA’s revenue in the October quarter, the company’s primary growth continues to come from its Data Center segment, driven by AI demand.
AI Advancements
NVIDIA introduced Nemotron, a new family of AI models derived from Meta’s Llama models, including Llama Nemotron Nano, Super, and Ultra, aimed at advancing AI agent capabilities. CEO Jensen Huang projects that the AI agent market could be worth trillions of dollars.
Additionally, NVIDIA confirmed that its Blackwell AI accelerators are in full production and are being adopted by leading cloud providers and PC manufacturers, further solidifying its position in AI technology.
Robotics and Autonomous Vehicles
NVIDIA debuted Cosmos, the "world's first physical AI model," designed to advance robotics. Trained on 20 million hours of video, Cosmos is open-licensed on GitHub and integrates seamlessly with NVIDIA’s Omniverse platform to provide physics-based simulations for AI model training in robotics and autonomous systems.
In partnership with Toyota, NVIDIA is collaborating on developing the automaker's latest autonomous vehicles. Huang sees robotics and autonomous technology as a \(\$1\) trillion market opportunity, expecting NVIDIA’s automotive revenue to grow from \(\$4\) billion in FY25 to \(\$5\) billion in FY26, spanning Data Center and OEM segments.
Project DIGITS
NVIDIA announced Project DIGITS, a personal AI supercomputer aimed at democratizing access to powerful AI tools. Starting at \(\$3,000\), the system features the GB10 Grace Blackwell Superchip, 128GB of unified memory, and up to 4TB of NVMe storage. Users can connect two systems for enhanced processing capabilities.
Designed for AI researchers and data scientists, Project DIGITS provides a cost-effective solution for building complex AI models without relying on large-scale data center resources.
A not comprehensive summary of NVIDIA's efforts on AI, not a summary of this YouTube video:
AI Compute Hardware:
This category includes the physical processing units that perform the core calculations for AI models. These are primarily GPUs, but also include specialized CPUs and other accelerators.
Focus: High-performance, parallel processing, low latency, memory bandwidth, energy efficiency for AI workloads.
Examples:
NVIDIA A100 Tensor Core GPU NVIDIA A40 Tensor Core GPU NVIDIA A10 Tensor Core GPU NVIDIA H100 Tensor Core GPU NVIDIA L40 GPU NVIDIA L4 GPU NVIDIA B100 "Blackwell" Data Center GPU NVIDIA Grace CPU Superchip GeForce RTX 30 Series (Desktop) - Ampere (Relevance for model development) GeForce RTX 50 Series (Desktop) - Blackwell (Relevance for model development) Project DIGITS - Hardware system (personal AI supercomputer).
AI Platforms & Systems:
This category includes integrated hardware and software solutions designed to simplify the development and deployment of AI applications. It encompasses both edge and data center solutions.
Focus: Ease of use, scalability, optimized performance for specific AI tasks, deployment solutions.
Examples:
NVIDIA DGX A100 System NVIDIA Jetson AGX Xavier NX NVIDIA Jetson Orin NVIDIA Jetson Orin Nano NVIDIA Omniverse Platform
AI Software & Development Tools:
This category includes the software libraries, frameworks, and tools that allow developers to build, train, and deploy AI models. It covers both open source and proprietary tools.
Focus: Developer productivity, model performance, framework support, customization.
Examples:
NVIDIA Merlin (Software Library) NVIDIA NeMo Framework NVIDIA TAO Toolkit
AI Applications & Solutions:
This category focuses on specific, industry-focused AI applications built on top of NVIDIA hardware and software.
Focus: Pre-built solutions, vertical market expertise, end-to-end solutions.
Examples: Intelligent Video Analytics (IVA), autonomous vehicle solutions, AI-driven healthcare, generative AI.
AI Research and Frameworks
While related to AI Software and development tools, it deserves its own category because of the open source nature of much of the research based tools and APIs, allowing for community contributions and new technology development.
Focus: Next-generation tools, advanced research, pushing the limits of AI, new technologies and algorythms.
Examples: Nemotron NVIDIA FLARE (Federated Learning Application Runtime Environment) NVIDIA Research Publications and Open-Source Projects TensorFlow and PyTorch (With NVIDIA's Extensions)
So, my takeaway was entirely different. It was not a commentary on Masa, or Larry, or Sam. I think all of those three companies are, frankly, very good. It was more a comment that you have to be very careful to protect the president's legacy, if I were them, to make sure that the things that get announced are actually further down the technical spectrum and are actually going to be real. Because if they achieve these things, but it costs you a billion dollars and you only hire 50 people, there's going to be a little bit of egg on the face. And so, that was sort of my own takeaway. I think that the things were decoupled. It just seemed more like marketing and sizzle and kind of hastily put together. I think it would be great if OpenAI builds another incredible model, whatever comes after o3, o4, o5. But it's not clear that you have to spend $500 billion to do it. - Chamath Palihapitiya
― Trump's First Week: Inauguration Recap, Executive Actions, TikTok, Stargate + Sacks is Back! - All-In Podcast [Link]
There's a thing called Jevons Paradox, which kind of speaks to this concept. SAA actually tweeted about it. It's an economic concept where, as the cost of a particular use goes down, the aggregate demand for all consumption of that thing goes up. So, the basic idea is that as the price of AI gets cheaper and cheaper, we're going to want to use more and more of it. You might actually get more spending on it in the aggregate. That's right—because more and more applications will become economically feasible. Exactly. That is, I think, a powerful argument for why companies are going to want to continue to innovate on frontier models. You guys are taking a very strong point of view that open source is definitely going to win, that the leading model companies are all going to get commoditized, and therefore, there will be no return on capital—essentially forcing continued innovation on the frontier. - David Sacks
But then there's this dark horse that nobody's talking about—it's called electricity. It's called power. And all these vehicles are electric vehicles. If you said, 'You know, I just did some quick back-of-the-envelope calculations,' if all of the miles in California went to EV ride-sharing, you would need to double the energy capacity of California. Right? Let's not even talk about what it would take to double the energy capacity of the grid and things like that in California. Let's not even go there. Even getting 10% or 20% more capacity is going to be a gargantuan, five-to-ten-year exercise. Look, I live in LA—in a nice area in LA—and we have power outages all the freaking time because the grid is messed up. They're sort of upgrading it as things break. That's literally where we're at in LA, in one of the most affluent neighborhoods. That’s just the reality. So, I think the dark horse, kind of hot take, is combustion engine AVs. Because I don’t know how you can scale AVs really, really massively with the electric grid as it is. - Travis Kalanick
I just wanted to read a message from Brian Yutko, who's the CEO of Wisk, which is building a lot of these autonomous systems. He said: 'First, automatic traffic collision avoidance systems do exist right now. These aircraft will not take control from the pilot to save the aircraft, even if the software and systems on the aircraft know that it’s going to collide. That’s the big flip that needs to happen in aviation—automation can actually kick in and take over, even in piloted aircraft, to prevent a crash. That’s the minimum of where we need to go. Some fighter jets have something called Automatic Ground Collision Avoidance Systems that do exactly this when fighter pilots pass out. It’s possible for commercial aviation as well.' And then, the second thing he said is: 'We need to have better ATC (Air Traffic Control) software and automation. Right now, we use VHF radio communications for safety and critical instructions, and that’s kind of insane. We should be using data links, etc. The whole ATC system runs on 1960s technology. They deserve better software and automation in the control towers—it’s totally ripe for change. The problem is that attempts at reform have failed.' - Chamath Palihapitiya
― DeepSeek Panic, US vs China, OpenAI $40B?, and Doge Delivers with Travis Kalanick and David Sacks - All-In Podcast [Link]
Articles and Blogs
The Art of Leading Teammates - Harvard Business Review [Link]
A Team-Focused Philosophy
- Put the team first, always, even when facing personal adversity.
- Show appreciation for unsung colleagues.
- Set the standard and create a culture of 100% effort.
- Recognize teammates’ individual psychology and the best ways to motivate them.
- Understand and complement the style of the formal leader.
- Recognize and counteract the external forces that can cause selfish behavior.
- Create opportunities to connect as people outside the office.
What Helps—and What Gets in the Way
- The emotions and behaviors that define individuals are formed early.
- Leaders work within a system.
- It can be hard for individual team leaders to influence change across large organizations.
- A leader’s style and influence will take time to evolve.
Early adopters of gen AI can eclipse rivals by using it to identify entirely new product opportunities, automate routine decisions and processes, deliver customized professional services, and communicate with customers more quickly and cheaply than was possible with human-driven processes.
Far from being a source of advantage, even in sectors where its impact will be profound, gen AI will be more likely to erode a competitive advantage than to confer one, because its very nature makes new insights and data patterns almost immediately transparent to anyone using gen AI tools.
If you already have a competitive advantage that rivals cannot replicate using gen AI, the technology may amplify the value you derive from that advantage.
Businesses that try to deny the power of gen AI will certainly fail. Those that adopt it will stay in the fight. But at this stage it looks likely that the only ones that will actually win with it will be those that can apply it to amplify the advantages they already have.
― AI Won’t Give You a New Sustainable Advantage - Harvard Business Review [Link]
To prevent this problem: Ask about this: Sample questions: Conflating Correlation And Causation Approach to determining causality Was this analysis based on an experiment? If not, are there confounders (variables that affect the independent and dependent variables)?To what extent were they addressed in the analysis? Misjudging The Potential Magnitude Of Effects Sample size and the precision of the results What was the average effect of the change? What was the sample size and the confidence interval (or range of likely values the true effect would fall into, and the degree to which one is certain it would fall into that range)? How would our course of action change, depending on where the true effect might lie? A Disconnect Between What Is Measured And What Matters Outcome measures What outcomes were measured? Were they broad enough? Did they capture key intended and unintended consequences? Were they tracked for an appropriate period of time? Were all relevant outcomes reported? How do we think they map to broader organizational goals? Misjudging Generalizability Empirical setting and subgroup analysis How similar is the setting of this study to our business context? Does the context or time period of the analysis make it more or less relevant to our decision? What is the composition of the sample being studied, and how does it influence the applicability of the results? Does the effect vary across subgroups or settings? Does this tell us anything about the generalizability of the results? Overweighting A Specific Result Broader evidence and further data collection Are there other analyses that validate the results and approach? What additional data could we collect, and would the benefit of gathering it outweigh the cost of collecting it? How might this change our interpretation of the results? ― Where Data-Driven Decision-Making Can Go Wrong - Harvard Business Review [Link]
Will Psychedelics Propel Your Career? - Harvard Business Review [Link]
Do you want to take a 'trip'? lol
How Scalable Compute Resources Can Boost LLM Performance - HuggingFace [Link]
This blog explains how to scale test-time compute for models like OpenAI's o1 - apply dynamic inference strategies to improve performance without increasing pretraining budgets. These techniques allow smaller models to outperform larger models on tasks such as math problems.
We introduce deliberative alignment, a training paradigm that directly teaches reasoning LLMs the text of human-written and interpretable safety specifications, and trains them to reason explicitly about these specifications before answering. We used deliberative alignment to align OpenAI’s o-series models, enabling them to use chain-of-thought (CoT) reasoning to reflect on user prompts, identify relevant text from OpenAI’s internal policies, and draft safer responses.
― Deliberative alignment: reasoning enables safer language models - OpenAI [Link]
Moravec’s paradox is the observation by artificial intelligence and robotics researchers that, contrary to traditional assumptions, reasoning requires very little computation, but sensorimotor and perception skills require enormous computational resources. The principle was articulated by Hans Moravec, Rodney Brooks, Marvin Minsky, and others in the 1980s.
― Common misconceptions about the complexity in robotics vs AI - Harimus Blog [Link]
Yes, as Yann LeCun mentioned in one of his previous campus lectures, LLM might help but it is not the right solution for robotics. This article made several good points:
- Sensorimotor Tasks Are More Complex. The source emphasizes that sensorimotor tasks are harder than many people realize. It was once assumed that perception and action were simple compared to reasoning, but this has turned out to be incorrect. This idea is known as Moravec's Paradox.
- Real-World Interaction is the challenge. Robotics requires robots to interact with a dynamic, chaotic, and complex real world. Tasks that seem simple for humans, like picking up a coffee cup, involve complex, unconscious processes that are hard to program for a robot. Even small changes in the environment can require a complete rewrite of the robot's "move commands". Robots need to break down movements into muscle contractions and forces, which is more complex than it seems.
- Data Requirements is another challenge. LLMs thrive on massive amounts of data, like text and images from the internet. Robotics requires precise, high-quality data that is hard to collect. The variety and preciseness of the data are also important. Unlike LLMs where quantity of data is key, in robotics, the quality of the data collected matters more than the quantity.
Regarding the question "do we need better hardware to learn", I think we need a system of sensors that can capture every physical movement of a body and every angle a body can perceive. In terms of a world model, the system needs to be on a larger scale.
OpenAI has created an AI model for longevity science - MIT Technology Review [Link]
OpenAI's success with GPT-4b micro demonstrates the potential of LLMs to go beyond natural language processing and address highly specialized scientific problems. The model's ability to redesign Yamanaka factors to improve their effectiveness by 50x could be a game-changer in stem cell research, accelerating advancements in regenerative medicine. This development highlights a significant milestone in the use of AI for scientific discovery, particularly in the field of protein engineering and regenerative medicine.
A classic pattern in technology economics, identified by Joel Spolsky, is layers of the stack attempting to become monopolies while turning other layers into perfectly-competitive markets which are commoditized, in order to harvest most of the consumer surplus; discussion and examples.
― Laws of Tech: Commoditize Your Complement - [Link]
This is exactly Meta's strategy initially competing with close-source AI model businesses - commoditize their complements to increase demand for their own products. And there are more examples mentioned in this article.
Core Concept:
Products have substitutes and complements. A substitute is an alternative product that can be bought if the first product is too expensive. A complement is a product usually bought together with another product. Demand for a product increases when the price of its complements decreases. Companies strategically try to commoditize their complements to increase demand for their own products. Commoditizing a complement means driving its price down to a point where many competitors offer indistinguishable goods. This strategy allows a company to become a quasi-monopolist and divert the majority of the consumer surplus to themselves.
How it works:
A company seeks a chokepoint or quasi-monopoly in a product composed of multiple layers. It dominates one layer of the stack while fostering competition in another layer. This drives down prices in the commoditized layer, increasing overall demand. The company profits from increased demand for its core product while the competitors in the commoditized layer struggle with low margins. The goal is to make a complement free or very cheap, to increase profits elsewhere. This strategy is an alternative to vertical integration.
Examples of Commoditization:
- Microsoft commoditized PC hardware by licensing its OS to many manufacturers, making the PC itself a commodity and increasing demand for MS-DOS.
- IBM commoditized the add-in market by using off-the-shelf parts and documenting the interfaces, allowing other manufacturers to produce add-on cards for their PCs, which increased the demand for PCs.
- Netscape open-sourced its web browser to commoditize browsers and increase demand for its server software.
- Various companies contribute to open-source software to commoditize software and increase demand for hardware and IT consulting services.
- Sun developed Java to make hardware more of a commodity.
- The Open Game License (OGL) was created to commoditize the Dungeons and Dragons system and drive sales of core rulebooks.
Open Source as a Strategic Weapon:
Open source can be a way for companies to commoditize their complements. It allows companies to share development costs and compete with dominant players. It can also neutralize advantages held by competitors and shift the focus of competition. Open sourcing can prevent a single company from locking up a technology.
Generalization:
Many products are composed of layers, each necessary but not sufficient for the final product. The final product is valuable, but the distribution of revenue among the different layers is contentious. Commoditizing complements is a way to control the market without vertical integration. The division of revenue is influenced by power plays and market dynamics.
Additional Examples:
The sources list many examples of commoditization in various industries, including hardware vs. software, banks vs. merchants, apps vs. OSes, game portals vs. game devs, telecom vs. users, and many more. The examples illustrate the breadth of this strategy across various tech and non-tech sectors. There are examples of companies commoditizing themselves, such as Stability AI, who commoditized image-generation models and saw little profit themselves.
Counter-Examples:
- Sun Microsystems' strategy of making both hardware and software a commodity was not successful.
- Some companies, like Apple, try to control both the hardware and software aspects of their products, which goes against the commoditization strategy.
Other Factors:
Antitrust actions can influence companies and prevent them from crushing competitors. Fear of antitrust actions may have stopped Microsoft from crushing Google.
Consequences:
The commoditization of complements can lead to intense competition in certain layers of the tech stack. It can also lead to a concentration of power and revenue in the hands of companies that control key chokepoints.
Reports and Papers
Mixtral of Experts [Link]
Key innovation: Sparse Mixture of Experts (SMoE) with TopK=2.
The state of Generative AI and Machine Learning at the end of 2023 - Intel Tiber AI Studio [Link]
Trends and insights of AI development and deployment in the enterprise - a survey result.
Does Prompt Formatting Have Any Impact on LLM Performance? [Link]
Prompt formats significantly affect LLM performance, with differences as high as 40% observed in code translation tasks for GPT-3.5-turbo. Larger models like GPT-4 demonstrate more resilience to prompt format changes.
JSON format outperformed Markdown in certain tasks, boosting accuracy by 42%. GPT-4 models exhibited higher consistency in responses across formats compared to GPT-3.5 models.
Deliberative Alignment: Reasoning Enables Safer Language Models [Link]
Their training methodology has two stages: 1) supervised fine-tuning on (prompt, CoT, output) datasets where CoTs explicitly reference safety policies, 2) high-compute RL using a reward model informed by safety policies, improving reasoning and adherence.
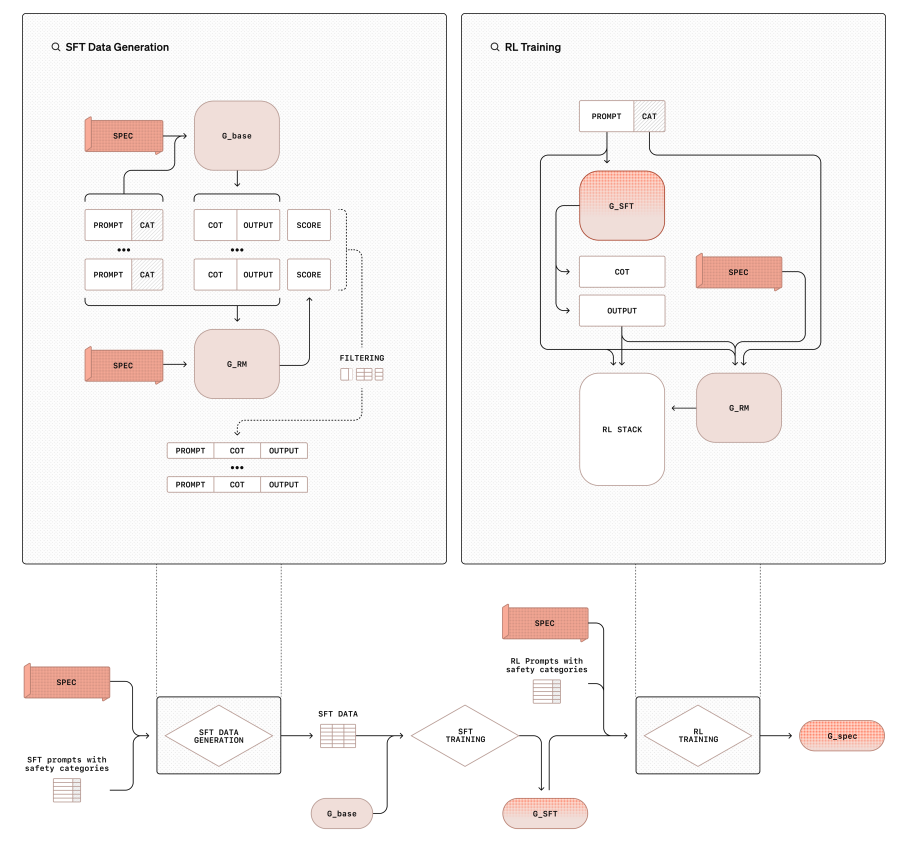
Genesis is a comprehensive physics simulation platform designed for general purpose Robotics, Embodied AI, & Physical AI applications. It is simultaneously multiple things:
- A universal physics engine re-built from the ground up, capable of simulating a wide range of materials and physical phenomena.
- A lightweight, ultra-fast, pythonic, and user-friendly robotics simulation platform.
- A powerful and fast photo-realistic rendering system.
- A generative data engine that transforms user-prompted natural language description into various modalities of data.
― Genesis: A Generative and Universal Physics Engine for Robotics and Beyond [Link]
Agents - Julia Wiesinger, Patrick Marlow, and Vladimir Vuskovic - Google [Link]
Agent AI: Surveying the Horizons of Multimodal Interaction [Link]
Foundations of Large Language Models [Link]
Atlas of Gray Matter Volume Differences Across Psychiatric Conditions: A Systematic Review With a Novel Meta-Analysis That Considers Co-Occurring Disorders [Link]
"Gray matter volume (GMV) differences across major mental disorders" refers to variations in the amount or density of gray matter in the brain when comparing individuals with mental disorders to those without. Gray matter consists of neuronal cell bodies, dendrites, and synapses and is essential for processing information, controlling movements, and supporting higher cognitive functions like memory, attention, and decision-making.
Structural Abnormalities: Mental disorders are often associated with changes in the brain's structure. GMV differences can highlight specific brain regions that are smaller, larger, or differently shaped in individuals with mental disorders.
Neurobiological Insights: Identifying GMV changes helps researchers understand the neurobiological basis of mental disorders and how these changes may contribute to symptoms like mood dysregulation, cognitive impairment, or altered behavior.
Target for Interventions: Understanding these differences can inform treatments such as targeted therapies, neurostimulation, or cognitive training to address the affected brain regions.
From Efficiency Gains to Rebound Effects: The Problem of Jevons’ Paradox in AI’s Polarized Environmental Debate [Link]
DeepSeek-R1: Incentivizing Reasoning Capability in LLMs via Reinforcement Learning [Link]
DeepSeek-R1 is an open-source reasoning model that matches OpenAI-o1 in math, reasoning, and code tasks.
News
NVIDIA Project DIGITS, A Grace Blackwell AI Supercomputer on your desk - NVIDIA [Link]
Constellation inks $1 billion deal to supply US government with nuclear power - Yahoo [Link]
Why 2025 will be the year of AI orchestration [Link]
2025 is anticipated to be the year of AI orchestration for several reasons:
- In 2024, there was broad experimentation in AI, particularly with agentic use cases. In 2025, these pilot programs, experiments, and new use cases are expected to converge, leading to a greater focus on return on investment.
- As organizations deploy more AI agents into their workflows, the need for infrastructure to manage them becomes more critical. This includes managing both internal workflows and those that interact with other services.
- Decision-makers, especially those outside of the technology sector, are seeking tangible results from their AI investments. They are moving beyond experimentation and expect to see a return on their investment in 2025.
- There will be a greater emphasis on productivity, which involves understanding how multiple agents can be made more effective. This will require a focus on accuracy and achieving higher productivity.
- Many new orchestration options are emerging to address the limitations of existing tools such as LangChain. Companies are building orchestration layers to manage AI applications. These frameworks are still early in development, and the field is expected to grow.
- There will be a focus on integrating agents across different systems and platforms, such as AWS's Bedrock and Slack, to allow for the transfer of context between platforms.
- The emergence of powerful reasoning models like OpenAI's o3 and Google's Gemini 2.0 will make orchestrator agents more powerful.
Perplexity AI makes a bid to merge with TikTok U.S. - CNBC [Link]
OpenAI, Alphabet Inc.’s Google, AI media company Moonvalley and several other AI companies are collectively paying hundreds of content creators for access to their unpublished videos, according to people familiar with the negotiations.
― YouTubers Are Selling Their Unused Video Footage to AI Companies - Bloomberg [Link]
Stavridis says Trump’s plan for Greenland ‘not a crazy idea’ - The Hill [Link]
California’s Wildfire Insurance Catastrophe - WSJ [Link]
Rising premiums and limited coverage options could significantly impact Californians, particularly in wildfire-prone areas. The article calls out state leadership for failing to adapt policies to address climate-related risks effectively.
“Our robotics team is focused on unlocking general-purpose robotics and pushing towards AG –level intelligence in dynamic, real-world settings. Working across the entire model stack, we integrate cutting-edge hardware and software to explore a broad range of robotic form factors. We strive to seamlessly blend high-level AI capabilities with the physical constraints of physical.“
― OpenAI has begun building out its robotics team - VentureBeat [Link]
It's surprising because I kind of remember in a public interview Sam said he is not going to go hardware as it's going to be as efficient on that as those companies with hardware foundations like Tesla, NVIDIA, Meta, etc. Now, it's hiring its first hardware robotics roles as announced by Caitlin Kalinowski.
OpenAI’s $500B ‘Stargate Project’ could aid Pentagon’s own AI efforts, official says - Breaking Defense [Link]
This article highlights OpenAI's ambitious Stargate Project and its potential impact on both commercial and government sectors, particularly the U.S. Department of Defense (DoD). Stargate represents a bold step in building the next generation of AI infrastructure, and its success could profoundly influence the future of both private AI development and national security capabilities. The collaboration between industry leaders and government stakeholders will be key to overcoming technical and financial hurdles.
Here are key takeaways:
OpenAI's Stargate Project:
- Objective: Build $500 billion worth of AI infrastructure, including new data centers and power solutions, primarily aimed at training and operating large AI models.
- Initial Funding: $100 billion to be deployed immediately, with ongoing development starting in Texas and other potential sites in the U.S.
- Collaborators: Japan-based SoftBank, Oracle, UAE-based MGX, NVIDIA, Microsoft, and Arm.
DoD Implications:
- AI Challenges in Defense: The DoD faces significant bottlenecks in computing power to meet the demands of modern AI applications, from battlefield decision-making to intelligence analysis and coordinating multi-domain operations (CJADC2).
- Reliance on Private Sector: Stargate could provide essential computing power to address the Pentagon's high-tech needs, especially where DoD lacks in-house capacity.
- Field Applications: Supercomputing resources are essential for training and retraining AI models in dynamic environments, such as battlefield conditions where new inputs may arise.
Challenges:
- Energy Demands: Generative AI models like ChatGPT consume immense electricity. The DoD must consider scalable and portable power sources, such as compact nuclear plants.
- Funding Scrutiny: Despite public commitments, concerns about the financial capability of Stargate’s backers, including SoftBank, have raised questions.
- Technical Constraints: Effective use of AI in military applications depends on robust, secure, and reliable infrastructure to handle high-bandwidth connections and avoid vulnerabilities to jamming.
Political and Economic Context:
- The Stargate Project was announced at a high-profile White House event, underscoring its perceived importance to national interests.
- Skepticism from figures like Elon Musk about the financial feasibility of such an enormous project adds to the intrigue surrounding its rollout.
Trump is planning 100 executive orders starting Day 1 on border, deportations and other priorities - AP News [Link]
A new neural-network architecture developed by researchers at Google might solve one of the great challenges for large language models (LLMs): extending their memory at inference time without exploding the costs of memory and compute. Called Titans, the architecture enables models to find and store during inference small bits of information that are important in long sequences.
Titans combines traditional LLM attention blocks with “neural memory” layers that enable models to handle both short- and long-term memory tasks efficiently. According to the researchers, LLMs that use neural long-term memory can scale to millions of tokens and outperform both classic LLMs and alternatives such as Mamba while having many fewer parameters.
― Google’s new neural-net LLM architecture separates memory components to control exploding costs of capacity and compute [Link]
TikTok restoring service after Trump vows to delay ban - AXIOS [Link]
TikTok's response to the Supreme Court decision [Link]
Amazon bought more renewable power last year than any other company - TechCrunch [Link]
Ozempic, Wegovy and other drugs are among 15 selected for Medicare’s price negotiations [Link]
Waymo Finds a Way Around US Restrictions Targeting Chinese Cars [Link]
More Speech and Fewer Mistakes - Meta News [Link]
NVIDIA Cosmos - NVIDIA [Link]
Announcing The Stargate Project - OpenAI [Link]
OpenAI announces the Stargate Project, a \(\$500\) billion effort to create advanced AI infrastructure. The project begins with an immediate \(\$100\) billion deployment for data centers, starting in Texas. It supports OpenAI’s goal of scaling artificial general intelligence (AGI) and training advanced AI models. Focus on high-value fields like personalized medicine and biotechnology.
NVIDIA GPUs power compute-intensive workloads. Oracle provides high-capacity cloud infrastructure. Microsoft Azure supports scalable distributed AI model training.
Introducing Operator - OpenAI [Link]
It's an AI agent that automates tasks directly in a web browser. You can use Operator to complete repetitive tasks like filling out forms, booking travel, or ordering items online. It uses a new model called Computer-Using Agent (CUA), which integrates GPT-4's vision capabilities with reinforcement learning to interact with graphical user interfaces (GUIs).
Introducing Citations on the Anthropic API - Anthropic [Link]