2025 February - What I Have Read
Substack
The key aspect of managing up is to learn to speak the language of your counterpart. If you can speak their language you can understand their goals and fears, and you can communicate at the level they are. You'll be in a better position to be an effective report. — Umberto Nicoletti, Head of R&D at Proemion
The better we understand the goals that our managers have, the less surprising their actions will be. […] Some of the situations where managers act in ways that most dismay or surprise us are when they are acting on their fears and worries. - Joe Chippindale, CTO Coach
― Frameworks for Managing Up as a Software Engineer - High Growth Engineer [Link]
Building Trust:
- Sincerity — you are honest and transparent, even when it’s uncomfortable. This includes admitting mistakes early, being upfront with challenges, and sharing both good and bad news, without sugar-coating the latter.
- Reliability — this is about consistency and following through. You do what you say you'll do, you set realistic expectations, and communicate proactively through regular update habits. More on this later in the updates section.
- Care — you have their best interests in mind. This means understanding their goals and challenges, being proactive in helping them succeed, and showing empathy when things get tough.
- Competence — finally, you deliver results. This goes beyond technical skills: it's about delivering business value, learning and growing from feedback, and understanding the big picture.
Speaking their language:
Map their context
- What makes you successful? — What are your goals and concerns?
- What makes me successful? — How can I help you reach your goals?
The only way for you to be successful is to make your manager successful. To do that, you need to be able to map your goals and concerns into their own.
Translate impact across altitudes
For any item you report to your manager, the question you should ask yourself is: why should my manager care about this? And, more subtly: what does my manager care about this?
Create explicit agreements
- Scope of ownership — do you know what decisions you can make autonomously vs when you need to involve your manager?
- Success criteria — how do you know if what you do is successful? Do you know how impact will be measured?
- Mutual expectations — do you know what your manager needs from you? And do they know what you need from them?
Creating effective updates
Define your update stack
- Async messages (daily) — about significant progress or blockers.
- Written reports (weekly) — structured updates about key results and next steps.
- 1:1s (weekly or biweekly) — deeper conversations about growth, wellbeing, and strategy.
Make every update count
- Why does this matter to my manager?
- What should they do with this information?
Build a feedback loop
Use 1:1s, retrospectives, and feedback moments to inspect your update process: what's working? What feels like noise? What critical information is missing?
JD Vance's AI Summit Paris Speech - Artificial Intelligence Survey [Link] [YouTube]
Here are some of JD Vance's main points regarding AI, on behalf of the Trump Administration:
- Vance emphasizes AI's potential for revolutionary applications and economic innovation and advocates against being too risk-averse. This is the main stance of this optimistic speech - more of AI opportunity, less of AI safety.
- He states the administration aims to ensure American AI technology remains the gold standard and the U.S. is the preferred partner for AI expansion. The U.S. wants to partner with other countries in the AI revolution with openness and collaboration, but this requires international regulatory regimes that foster creation rather than strangling it.
- He expresses concern that excessive regulation could stifle the AI industry and supports a deregulatory approach. He mentions the development of an AI action plan that avoids overly precautionary regulatory regimes, while ensuring that all Americans benefit from the technology and its transformative potential. The administration is troubled by foreign governments tightening regulations on U.S. tech companies with international footprints. Vance states that preserving an open regulatory environment has encouraged American innovators to experiment.
- He stresses that American AI should not be co-opted for authoritarian censorship and should be free from ideological bias.
- He notes the importance of building the most powerful AI systems in the U.S. with American-designed and manufactured chips.
- He believes AI should be a tool for job creation and making workers more productive, prosperous, and free. The administration will always center American workers in its AI policy and ensure that AI makes workers more productive. For all major AI policy decisions coming from the federal government, the Trump Administration will guarantee American workers a seat at the table.
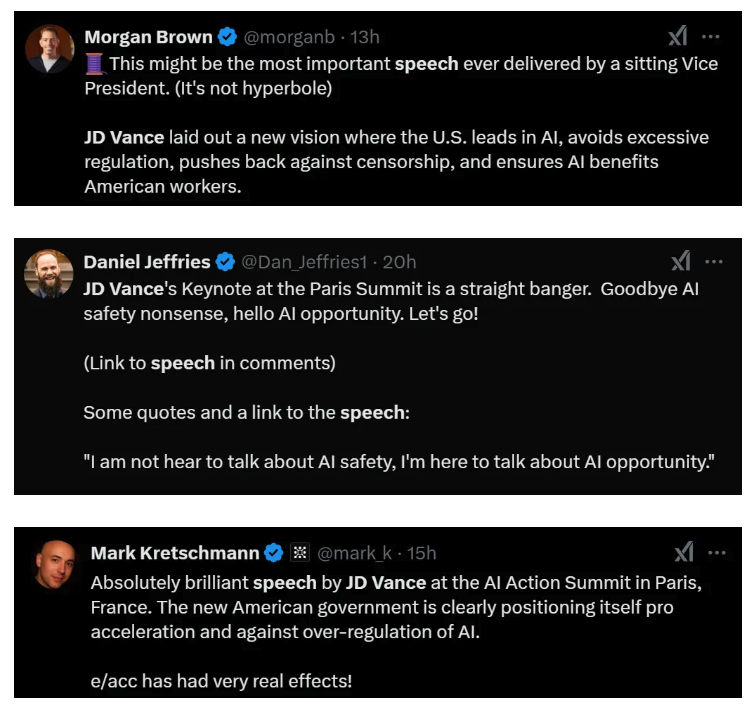
Elon Musk Blocked a Bill to Stop Amazon from Helping Kids Kill Themselves - BIG by Matt Stoller [Link]
In December, Elon Musk pushed for the reduction of government funding legislation, which led to the removal of several provisions. One provision removed due to Musk's intervention was the Youth Poisoning Prevention Act, which would have prevented consumers from buying concentrated sodium nitrite, a chemical often used in teenage suicides. This chemical, while used in low concentrations as a food preservative, is lethal in high concentrations and has no household uses.
The article highlights that Musk, who has significant political power, can make harmful mistakes, sometimes unknowingly. The author notes that the removal of the provision was considered a mistake that could be fixed. Despite bipartisan support for the priorities, there has been no action taken to reinstate them. He questions whether anyone will address and rectify the issues that arise from actions taken by figures like Musk and Trump.
Deep Research, information vs. insight, and the nature of science - Interconnects [Link]
This is a very interesting point: the article considers how AI might challenge Thomas Kuhn's theories of scientific revolutions. Kuhn's The Structure of Scientific Revolutions describes how science evolves, with scientists forming paradigms around theories and using them to gain knowledge until limitations necessitate a new paradigm. Here's how AI might challenge Kuhn's theories:
- AI is accelerating scientific progress, potentially faster than paradigms can be established. The fundamental unit of scientific progress is reducing so quickly that it redefines experimentation methods.
- Kuhn emphasizes that scientific knowledge is a process, not a set of fixed ideas. AI's emergence challenges this.
- Kuhn suggests science is done by a community that slowly builds out the frontier of knowledge, rather than filling in a known space. The article questions how the dynamics of science will change with AI systems.
- Kuhn states that to reject one paradigm requires the simultaneous substitution of another. The article implies that AI's rapid advancements may disrupt this process.
Check out this impressive list of stories they’ve broken since Trump took office:
- Elon Musk Plays DOGE Ball—and Hits America’s Geek Squad (Steven Levy, 1/22/25)
- DOGE Will Allow Elon Musk to Surveil the US Government From the Inside (Vittoria Elliott, 1/24/25)
- Elon Musk Lackeys Have Taken Over the Office of Personnel Management (Vittoria Elliott, 1/28/25)
- Elon Musk Is Running the Twitter Playbook on the Federal Government (Zoë Schiffer, 1/28/25)
- Elon Musk Tells Friends He’s Sleeping at the DOGE Offices in DC (Zoë Schiffer, 1/29/25)
- Government Tech Workers Forced to Defend Projects to Random Elon Musk Bros (Makena Kelly, Vittoria Elliott, 1/30/25)
- Elon Musk’s Friends Have Infiltrated Another Government Agency (Makena Kelly, Zoë Schiffer, 1/31/25)
- US Government Websites Are Disappearing in Real Time (Vittoria Elliott, Dhruv Mehrotra, Dell Cameron, 2/1/25)
- DOGE Staff Had Questions About the ‘Resign’ Email. Their New HR Chief Dodged Them (Makena Kelly, 2/1/25)
- The Young, Inexperienced Engineers Aiding Elon Musk’s Government Takeover (Vittoria Elliott, 2/2/25)
- Pronouns Are Being Forcibly Removed From Government Signatures (Dell Cameron, 2/3/25)
- Elon Musk Ally Tells Staff ‘AI-First’ Is the Future of Key Government Agency (Makena Kelly, 2/3/25)
- Elon Musk’s DOGE Is Still Blocking HIV/AIDS Relief Exempted From Foreign Aid Cuts (Kate Knibbs, 2/3/25)
- A 25-Year-Old With Elon Musk Ties Has Direct Access to the Federal Payment System (Vittoria Elliott, Dhruv Mehrotra, Leah Feiger, Tim Marchman, 2/4/25)
- Federal Workers Sue to Disconnect DOGE Server (Dell Cameron, 2/4/25)
- State Department Puts ‘All Direct Hire’ USAID Personnel on Administrative Leave (Kate Knibbs, 2/4/25)
- Elon Musk’s Takeover Is Causing Rifts in Donald Trump’s Inner Circle (Jake Lahut, 2/5/25)
When It Comes to Covering Musk's Government Takeover, WIRED Is Showing Everyone How It's Done - The Present Age [Link]
The Media Is Missing the Story: Elon Musk Is Staging a Coup - The Present Age [Link]
Mainstream media is refusing to tell the truth. WIRED deserves support.
"Character Limit - How Elon Musk Destroyed Twitter" by Kate Conger and Ryan Mac, as a reference.
2025: the Year of Datacenter Mania - AI Supremacy [Link]
This is an overview of what's happening and going to happen around Data Center Construction, covering a wide range of areas.
AI Expansion and Energy Demand:
The AI race is intensifying, leading to significant capital expenditure by Big Tech and raising concerns about potential harmful consequences. AI data centers' power demands are rapidly increasing, with estimates of needing 10 gigawatts of additional capacity in 2025 alone.
Goldman Sachs Research projects a 165% increase in data center power demand by 2030. By 2027, global AI data center power demand could reach 68 GW and 327 GW by 2030, compared to a total global data center capacity of 88 GW in 2022. Training AI models could require up to 1 GW in a single location by 2028 and 8 GW by 2030.
Infrastructure and Logistical Challenges:
Power infrastructure delays are increasing wait times for grid connections, which can take four to seven years in key regions. Data centers face struggles with local and state permits, especially for backup generators and environmental impact assessments.
A lack of data center infrastructure in the U.S. could cause a shift of construction to other countries. Countries with greater compute access may gain economic and military advantages.
Environmental and Health Concerns:
There are growing concerns that the impact of data centers on human health is being overlooked, and one of President Biden's executive orders acknowledges that data centers are harmful to health.
The environmental cost of AI includes concerns about water consumption, air pollution, electronic waste, and critical materials, in addition to public health concerns around pollution.
Energy Solutions and the Nuclear Option:
To meet AI’s growing power needs, some experts advocate for nuclear energy as the most viable long-term solution. Nuclear energy produces no carbon emissions during operation and offers a reliable, constant energy supply. Tech giants like Microsoft and Google are recognizing nuclear energy’s potential, with Microsoft exploring small modular reactors (SMRs). The adoption of nuclear energy faces obstacles such as high upfront costs, regulatory hurdles, and public skepticism.
Global AI Race and Investments:
The EU is mobilizing $200 billion in AI investments, signifying a global race for AI leadership. The UAE is investing billions in AI data centers in France and is implicated, along with SoftBank and Oracle, in OpenAI's data center project in Abilene, Texas.
The Question of Sustainability:
AI's rapid expansion is testing the limits of power infrastructure, natural resources, and sustainability efforts. If AI continues to expand at its current rate, there is a risk of a gridlocked future limited by energy availability. The future of AI depends on sustainability and the willingness to sacrifice energy for intelligence.
Amazon: Outspending Everyone - App Economy Insights [Link]
Some highlights:
- Amazon is planning to invest over $100 billion in 2025, primarily in AI-related infrastructure. This is more than any other company, and a 20% increase from 2024.
- AWS revenue grew 19% Y/Y, and roughly half of the growth is attributed to AI. AWS has a 30% market share in cloud infrastructure. Amazon is focused on custom silicon (Trainium and Inferentia) to improve AI efficiency.
- The de minimis exemption, which allows imports under $800 to avoid US tariffs, gives companies like Shein and Temu a competitive edge. Amazon Haul was launched last year to compete directly with these companies. Should the de minimis loophole be eliminated, Amazon's superior logistics network could give it an advantage in fulfillment and reliability.
- Amazon Prime's multi-faceted membership is highly effective at reducing churn. A 2022 study by the National Research Group found that Prime has one of the lowest churn rates, second only to cloud storage and music streaming services. Amazon's detailed purchase data provides advertisers with a valuable advantage, enabling highly targeted CTV ads with industry-leading returns on ad spend (ROAS).
Uber’s Three-Pronged AV Strategy:
- Fleet partnerships: Uber isn’t building its own AVs. Instead, it partners with companies like Waymo, Motional, and Aurora, integrating their fleets into Uber’s network.
- Hybrid model: AVs can’t handle all trips—human drivers will fill gaps, handling extreme weather, complex routes, and peak hours for decades.
- Fleet infrastructure: Uber is investing in charging depots and fleet management to maximize AV asset utilization.
While Tesla is vertically integrated, its rideshare strategy may take a different path. If Tesla adopts an asset-light model, Tesla owners—not Tesla itself—would decide whether to list their AVs on Uber. If maximum utilization is the goal, Uber could be the logical choice.
When it comes to demand aggregation, Uber remains the undisputed leader—its network effects ensure that as long as it aggregates supply, demand will follow, and gross profit will scale.
While the rideshare market will become more fragmented, Uber could still be the biggest fish in a much larger pond. After all, Uber is already the Airbnb for cars.
Tesla has a massive opportunity once the pieces fall into place. But with auto sales under pressure and market share declining, it still faces a long road ahead before claiming the top spot in any market.
― Tesla vs. Uber: Collision Course? - App Economy Insights [Link]
Uber's business model is one of my favorite business models. Not only because it's asset-light, its network effect, etc, but also because it created millions of jobs.
Uber's AV strategy is designed to balance innovation with practicality, ensuring that the company remains competitive while minimizing risks and costs. By leveraging partnerships, maintaining a hybrid model, and investing in infrastructure, Uber is well-positioned to lead the transition to autonomous mobility.
While a partnership between Uber and Tesla is possible and could offer significant synergies, it is not guaranteed. The decision would depend on whether both companies can align their goals and overcome competitive tensions. If Tesla decides to prioritize its own ride-hailing network (Tesla Network), it may choose to compete rather than collaborate with Uber. However, if Tesla sees more value in leveraging Uber’s platform and customer base, a partnership could be a strategic move for both companies.
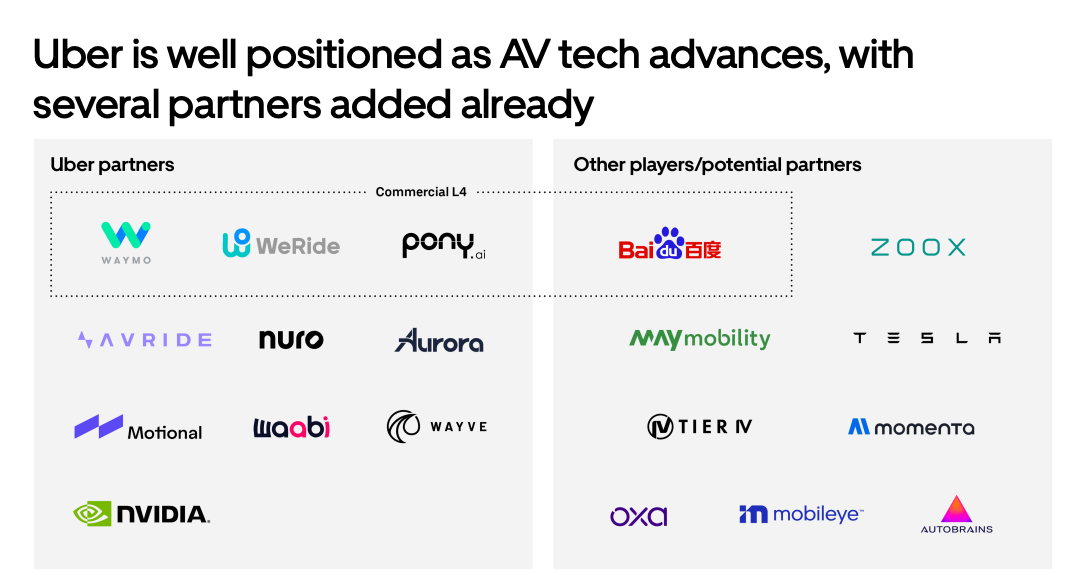
Microsoft: AI Efficiency Paradox - App Economy Insights [Link]
Google: Capex Arms Race - App Economy Insights [Link]
The End of Search, The Beginning of Research - One Useful Thing [Link]
Huang’s take: “We've really only tapped consumer AI and search and some amount of consumer generative AI, advertising, recommenders, kind of the early days of software. […] Future reasoning models can consume much more compute.”
DeepSeek-R1, he said, has “ignited global enthusiasm” and will push reasoning AI into even more compute-intensive applications.
― NVIDIA: AI's 3 Scaling Laws - App Economy Insights [Link]
Huang introduced a framework for AI’s evolving compute demands, outlining three scaling laws:
- Pre-training scaling: Traditional model growth through data consumption, now enhanced by multimodal learning and reasoning-based data.
- Post-training scaling: The fastest-growing compute demand, driven by reinforcement learning from human and AI feedback. This phase now exceeds pre-training in compute usage due to the generation of synthetic data.
- Inference & reasoning scaling: The next major shift, where AI engages in complex reasoning (e.g., chain-of-thought, search). Inference already requires 100x more compute than early LLMs and could scale to millions of times more.
Jensen Huang outlined a three-layer AI transformation across industries:
- Agentic AI (Enterprise AI): AI copilots and automation tools boosting productivity in sectors like automotive, finance, and healthcare.
- Physical AI (AI for Machines): AI-driven training systems for robotics, warehouses, and autonomous vehicles.
- Robotic AI (AI in the Real World): AI enabling real-world interaction and navigation, from self-driving cars to industrial robots.
Grab: The Uber Slayer - App Economy Insights [Link]
DeepSeek isn’t a threat—it’s validation. If AI inference costs are falling, Meta stands to benefit more than almost any other company. Instead of challenging its strategy, DeepSeek reinforces that heavy AI investments will pay off—not the other way around.
― Meta: DeepSeek Tailwinds - App Economy Insights [Link]
Elon Musk and spiky intelligence - Silver Bulletin [Link]
Interesting study on spiky intelligence, using Elon as a case study. Concepts highlights:
Spiky Intelligence: This refers to individuals who exhibit exceptional abilities in certain areas while being deficient in others. It contrasts with the idea of general intelligence (the "g factor"), where most cognitive abilities are positively correlated. Spiky intelligence is often seen in people who excel in abstract, analytical reasoning but may lack emotional intelligence, empathy, or practical judgment.
Berkson’s Paradox: This statistical phenomenon explains why successful individuals often appear to have significant weaknesses. In highly competitive fields, it’s rare to find people who excel in all dimensions, so success often goes to those with a few standout traits.
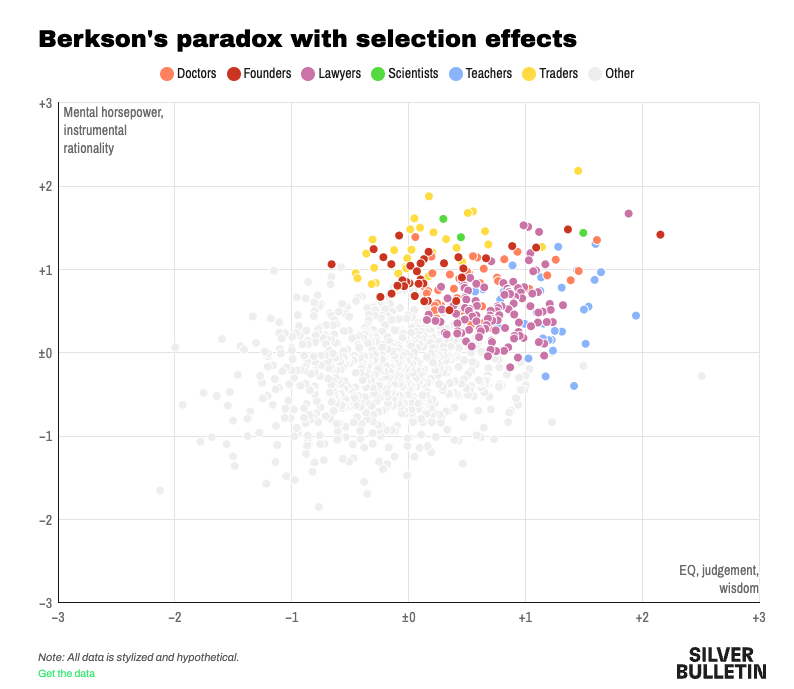
YouTube and Podcasts
DOGE vs USAID, Crypto Framework, Google's $75B AI Spend, US Sovereign Wealth Fund, GLP-1s - All-In Podcast [Link]
DeepSeek, China, OpenAI, NVIDIA, xAI, TSMC, Stargate, and AI Megaclusters - Lex Fridman Podcast #459 [Link] [Transcript]
This is a very good one. 5 hours intro and overview of current AI landscape.
Those driver jobs weren't even there 10 years ago. Uber came along and created all these driver jobs. DoorDash created all these driver jobs. So what technology does—yes, technology destroys jobs—but it replaces them with opportunities that are even better. And then, either you can go capture that opportunity yourself, or an entrepreneur will come along and create something that allows you to capture those opportunities. AI is a productivity tool. It increases the productivity of a worker; it allows them to do more creative work and less repetitive work. As such, it makes them more valuable. Yes, there is some retraining involved, but not a lot. These are natural language computers—you can talk to them in plain English, and they talk back to you in plain English. But I think David is absolutely right. I think we will see job creation by AI that will be as fast or faster than job destruction. You saw this even with the internet. Like, YouTube came along—look at all these YouTube streamers and influencers. That didn’t used to be a job. New jobs—really, opportunities—because 'job' is the wrong word. 'Job' implies someone else has to give it to me, like they're handed out, as if it's a zero-sum game. Forget all that—it's opportunities. After COVID, look at how many people are making money by working from home in mysterious little ways on the internet that you can't even quite grasp. - Naval Ravikant
you know as long as you remain adaptive and you keep learning and you learn how to take advantage of these tools you should do better and if you wall yourself off from the technology and don't take advantage of it that's when you put yourself at risk - David Sacks
If you trained on the open web, your model should be open source. – Naval Ravikant.
To keep the conversation moving, let me segue a point that came up that was really important into tariffs. And the point is, even though the internet was open, the U.S. won a lot of the internet—a lot of U.S. companies won the internet. And they won that because we got there "the firstest with the mostest," as they say in the military. And that matters because a lot of technology businesses have scale economies and network effects underneath, even basic brand-based network effects. If you go back to the late '90s and early 2000s, very few people would have predicted that we would have ended up with Amazon basically owning all of e-commerce. You would have thought it would have been perfect competition and very spread out. And that applies to how we end up with Uber as basically one taxi service or how we end up with Airbnb. Meta—Airbnb—it's just network effects, network effects, network effects rule the world around me. But when it comes to tariffs and when it comes to trade, we act like network effects don't exist. The classic Ricardian comparative advantage dogma says that you should produce what you're best at, I produce what I'm best at, and we trade. And then, even if you want to charge me more for it—if you want to impose tariffs for me to ship to you—I should still keep tariffs down because I'm better off. You're just selling me stuff cheaply—great. Or if you want to subsidize your guys—great, you're selling me stuff cheaply. The problem is, that is not how most modern businesses work. Most modern businesses have network effects. As a simple thought experiment, suppose that we have two countries, right? I'm China, you're the U.S. I start out by subsidizing all of my companies and industries that have network effects. So I'll subsidize TikTok, I'll ban your social media but push mine. I will subsidize my semiconductors, which tend to have winner-take-all dynamics in certain categories. Or I'll subsidize my drones and then, exactly—BYD, self-driving, whatever. And then, when I win, I own the whole market and I can raise prices. And if you try to start up a competitor, it's too late—I've got network effects. Or if I've got scale economies, I can lower my price to zero, crash you out of business, no one in their right mind will invest, and then I'll raise prices right back up. So you have to understand that certain industries have hysteresis, or they have network effects, or they have economies of scale—and these are all the interesting ones. These are all the high-margin businesses. So in those, if somebody is subsidizing or they're raising tariffs against you to protect their industries and let them develop, you do have to do something. You can't just completely back down. - Naval Ravikant
I think Sam and his team would do better to leave the nonprofit part alone, leave an actual independent nonprofit board in charge, and then have a strong incentive plan and a strong fundraising plan for the investors and the employees. So I think this is workable. It's just that trying to grab it all seems way off, especially when it was built on open algorithms from Google, open data from the web, and nonprofit funding from Elon and others. - Naval Ravikant
― JD Vance's AI Speech, Techno-Optimists vs Doomers, Tariffs, AI Court Cases with Naval Ravikant - All-In Podcast [Link]
"AI won't take your job; it's someone using AI that will take your job." – Richard Baldwin. The discussion around AI's impact on jobs is often framed as a zero-sum game, but the reality is more nuanced. While AI will displace certain jobs (e.g., self-driving cars replacing drivers), it will also create new opportunities and industries that we can't yet fully envision. The key is adaptability—those who learn to use AI tools will thrive, while those who resist will fall behind.
The Stablecoin Future, Milei's Memecoin, DOGE for the DoD, Grok 3, Why Stripe Stays Private - All-In Podcast [Link]
How to build full-stack apps with OpenAI o1 pro - Part 1 - Mckay Wrigley [Link]
Learn app development using OpenAI o1-Pro with a structured six-prompt workflow.
Open Deep Research - LangChain [Link]
Build and run a deep research agent with LangGraph Studio, customize configurations, compare architectures, and analyze costs.
Paper and Reports
Probabilistic weather forecasting with machine learning [Link]
GenCast's success stems from its ability to generate ensembles of sharp, realistic weather trajectories and well-calibrated probability distributions.
The methodology of GenCast involves several key components:
- GenCast employs a second-order Markov assumption, meaning it conditions its predictions on the two previous weather states, rather than just one. This is done because conditioning on two previous time steps works better than one.
- GenCast is implemented as a conditional diffusion model. Diffusion models are generative machine learning methods that can model the probability distribution of complex data and generate new samples by iteratively refining a noisy initial state. The model predicts a residual with respect to the most recent weather state. The sampling process begins with random noise, which is then refined over a series of steps.
- At each step of the iterative refinement process, GenCast uses a denoiser neural network. This network is trained to remove noise that has been artificially added to atmospheric states. The architecture of the denoiser includes an encoder, a processor, and a decoder. The encoder maps the noisy target state to an internal representation on a refined icosahedral mesh, the processor is a graph transformer, and the decoder maps the internal mesh representation back to a denoised target state.
- GenCast uses a noise distribution that respects the spherical geometry of global weather variables. Rather than using independent and identically distributed (i.i.d.) Gaussian noise on the latitude-longitude grid, it samples isotropic Gaussian white noise on the sphere and projects it onto the grid.
- GenCast's performance is evaluated using various metrics, including:
- CRPS (Continuous Ranked Probability Score): Measures the skill of a probabilistic forecast.
- RMSE (Root Mean Squared Error): Measures how closely the mean of an ensemble of forecasts matches the ground truth.
- Spread/Skill Ratios and Rank Histograms: Used to evaluate the calibration of the forecast distributions.
- Brier Skill Score: Evaluates probabilistic forecasts of binary events, specifically the prediction of extreme weather events.
- Relative Economic Value (REV): Characterizes the potential value of a forecast over a range of probability decision thresholds.
- Spatially Pooled CRPS: Evaluates forecasts aggregated over circular spatial regions of varying sizes to assess the model's ability to capture spatial dependencies.
- Regional Wind Power Forecasting: Evaluates the model's ability to predict wind power generation at wind farm locations using a standard idealized power curve.
- Tropical Cyclone Track Prediction: Uses the TempestExtremes tropical cyclone tracker to extract cyclone trajectories from the forecast and analysis data. The model's ability to forecast cyclone tracks is evaluated using position error and track probability.
The United States currently leads the world in data centers and AI compute, but unprecedented demand leaves the industry struggling to find the power capacity needed for rapidly building new data centers. Failure to address current bottlenecks may compel U.S. companies to relocate AI infrastructure abroad, potentially compromising the U.S. competitive advantage in compute and AI and increasing the risk of intellectual property theft.
― AI's Power Requirements Under Exponential Growth - RAND [Link] [pdf]
Genome modeling and design across all domains of life with Evo 2 - Arc Institute [Link]
Evo 2 is a powerful genome modeling and design tool that operates across all domains of life. It can analyze and generate genetic sequences from molecular to genome scale. It accurately assigns likelihood scores to human disease variants, distinguishing between pathogenic and benign mutations in both coding and noncoding regions. It can predict whether genes are essential or nonessential using mutational likelihoods, helping in bacterial and phage gene essentiality studies. It can generate large-scale DNA sequences with structured features like tRNAs, promoters, and genes with intronic structures. It provides zero-shot fitness predictions for protein and non-coding RNA sequences, correlating well with experimental fitness measurements. It robustly predicts the pathogenicity of various mutation types, achieving state-of-the-art performance for noncoding and splice variants.
Large Action Models: From Inception to Implementation [Link]
Microsoft Research published one of the most complete papers in this area, outlining a complete framework for large action models (LAMs) models. The core idea is to bridge the gap between the language understanding capability of LLMs and the need for real-world action execution.
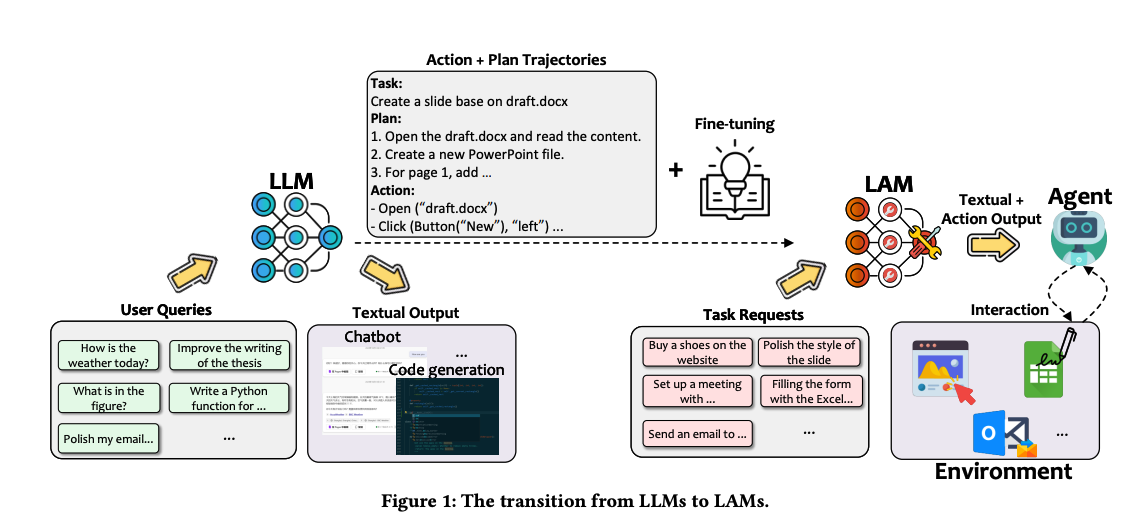
Towards an AI co-scientist [Link]
Uncovering the Impact of Chain-of-Thought Reasoning for Direct Preference Optimization: Lessons from Text-to-SQL [Link]
Direct Preference Optimization (DPO) does not consistently improve performance in the Text-to-SQL task and sometimes even degrades it. Existing Standard Fine-Tuning (SFT) methods are limited by the lack of high-quality training data, and prompting-based methods are expensive, slow, and raise data privacy concerns.
To solve the problems, they generate synthetic CoT solutions to improve training datasets, leading to more stable and significant performance improvements in DPO. They integrate execution-based feedback to refine the model’s SQL generation process, making the optimization process more reliable. And they create a quadruple-based preference dataset to help the model learn to distinguish between correct and incorrect SQL responses more effectively.
MONA: Myopic Optimization with Non-myopic Approval Can Mitigate Multi-step Reward Hacking [Link]
Google DeepMind developed an innovative approach - Myopic Optimization with Non-myopic Approval (MONA), to mitigate multi-step reward hacking. This MONA methodology is built on two key principles. The first is myopic optimization, where agents focus on maximizing rewards for immediate actions rather than planning multi-step strategies. This ensures that agents do not develop complex, unintelligible tactics. The second principle is non-myopic approval, where human overseers assess the agent's actions based on their expected long-term utility. These evaluations serve as the primary mechanism for guiding agents toward behavior aligned with human-defined objectives, without relying on direct feedback from outcomes.
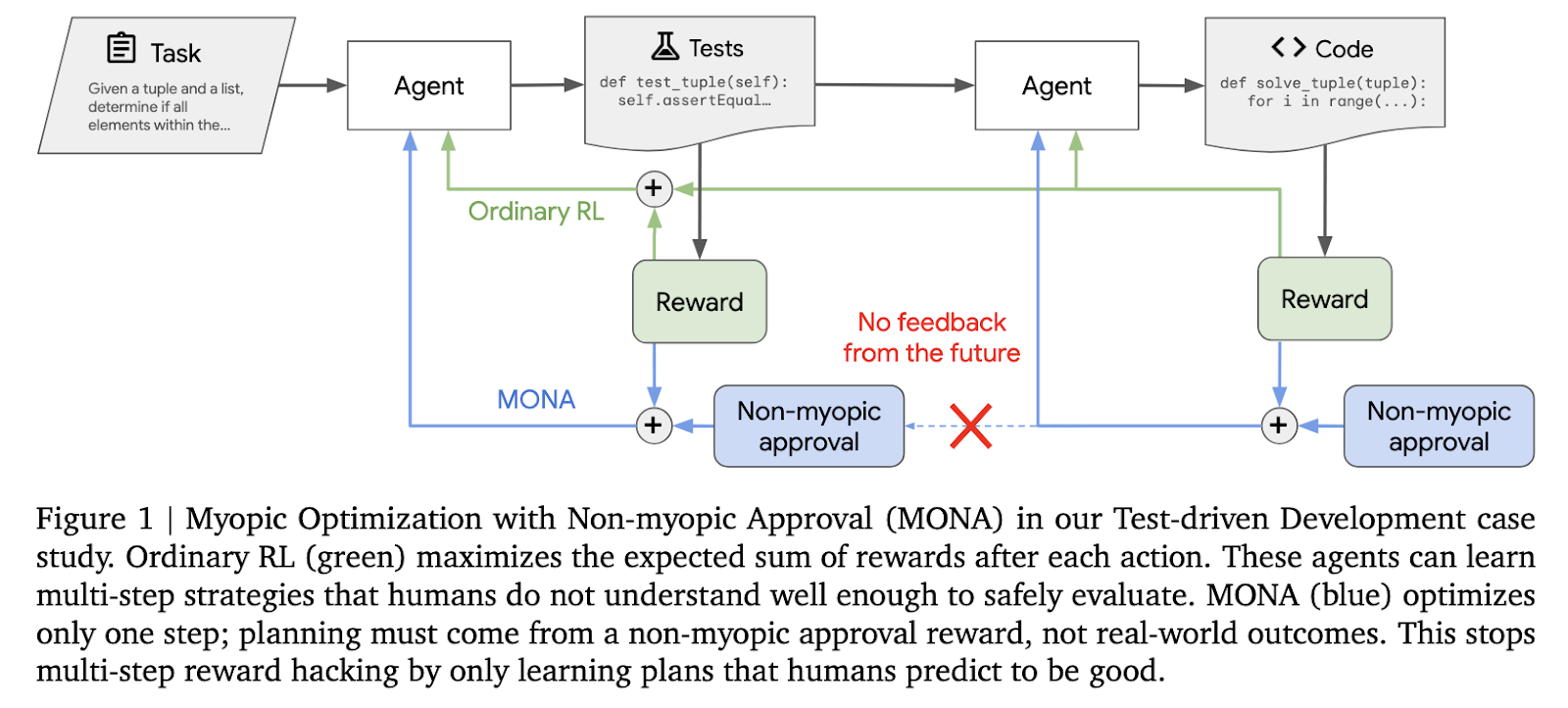
The Ultra-Scale Playbook: Training LLMs on GPU Clusters - Hugging Face [Link]
This book from Hugging Face explains 5D parallelism, ZeRO, CUDA kernel optimizations, and compute-communication overlap in large-scale AI training. It breaks down scaling bottlenecks, PyTorch internals, and parallelism techniques like ZeRO-3, pipeline, sequence, and context parallelism.
Articles and Blogs
The research found six distinct leadership styles, each springing from different components of emotional intelligence. The styles, taken individually, appear to have a direct and unique impact on the working atmosphere of a company, division, or team, and in turn, on its financial performance. And perhaps most important, the research indicates that leaders with the best results do not rely on only one leadership style; they use most of them in a given week—seamlessly and in different measure—depending on the business situation. Imagine the styles, then, as the array of clubs in a golf pro’s bag. Over the course of a game, the pro picks and chooses clubs based on the demands of the shot. Sometimes he has to ponder his selection, but usually it is automatic. The pro senses the challenge ahead, swiftly pulls out the right tool, and elegantly puts it to work. That’s how high-impact leaders operate, too.
Leaders who have mastered four or more—especially the authoritative, democratic, affiliative, and coaching styles—have the very best climate and business performance.
The leader can build a team with members who employ styles she lacks.
― Leadership That Gets Results - Harvard Business Review [Link]
WeatherNext - Google DeepMind [Link]
GenCast predicts weather and the risks of extreme conditions with state-of-the-art accuracy - Google DeepMind [Link]
Morgan Stanley stated that ASICs perform exceptionally well in certain specific application scenarios, but are highly dependent on the custom needs of particular clients; the development cost of ASICs is usually lower, but their system costs and Software deployment costs may be much higher than GPUs that can be commercially scaled, leading to a higher total cost of ownership. In addition, NVIDIA's CUDA ecosystem is very mature and widely used in Global Cloud Computing Services, with a market position that remains as solid as ever.
― Morgan Stanley: ASICs are overheated, and NVIDIA's position is difficult to shake. - moomoo [Link]
NVIDIA possesses a robust competitive advantage in the AI chip market due to its mature ecosystem, continuous R&D investments, and strong technical capabilities.
- NVIDIA's CUDA ecosystem is well-established, enabling clients to easily deploy and run various workloads. The maturity of this ecosystem means that customers may find it easier to use NVIDIA products compared to adapting software for ASICs or other alternatives.
- NVIDIA has a leading position in the AI chip market, which is reinforced by its presence on every cloud platform across the globe. Investments within NVIDIA's ecosystem benefit from global dissemination, further solidifying its market dominance.
- NVIDIA invests significantly in R&D. The company is expected to invest approximately \(\$16\) billion in R&D this year. This level of investment allows NVIDIA to maintain a 4-5 year development cycle and continuously introduce leading high-performance chips. Custom ASIC development budgets are typically smaller (less than \(\$1\) billion), giving NVIDIA an edge in innovation.
- NVIDIA is difficult to surpass in providing high-end training capabilities. The company focuses on training multi-modal AGI models.
This means DeepResearch can identify cross-domain links or examples that might otherwise be overlooked, offering fresh perspectives. In professional settings, this can support more well-rounded decision-making – for example, a product manager can quickly gather insights from scientific research, market data, and consumer opinions in one place, rather than relying on multiple teams or lengthy research processes. It makes you multifaceted!
― #87: Why DeepResearch Should Be Your New Hire - Turing Post [Link]
Deep Research and Knowledge Value - Stratechery [Link]
OpenAI launched Deep Research in ChatGPT, which is an agentic capability that conducts multi-step research on the internet for complex tasks. It synthesizes knowledge in an economically valuable way but does not create new knowledge.
As demonstrated in the article, it can be useful for researching people and companies before conducting interviews. However, it can also produce reports that are completely wrong by missing major entities in an industry.
This is a good point - The Internet revealed that news was worthless in terms of economic value because the societal value does not translate to economic value. Deep Research reveals how much more could be known, but the increasing amount of "slop" makes it more difficult to find the right information. Information that matters and is not on the Internet has future economic value wrapped up in it.
Proprietary data is valuable, and AI tools like Deep Research make it more difficult to harvest alpha from reading financial filings. Prediction markets may become more important as AI increases the incentive to keep things secret.
As a summary of the impact - Deep Research is a good value, but it is limited by the quality of information on the Internet and the quality of the prompt. There is value in the search for and sifting of information, and this may be lost with on-demand reports. AI will replace knowledge work. Secrecy is a form of friction that imposes scarcity on valuable knowledge. Deep Research is not yet good at understanding some things.
Massive Foundation Model for Biomolecular Sciences Now Available via NVIDIA BioNeMo - NVIDIA Blog [Link]
Grok 3: Intelligence, Performance & Price Analysis - Artificial Analysis [Link]
Grok resets the AI race - The Verge [link]
Grok-3 (chocolate) is the first-ever model to break 1400 score and is now #1 in Arena.
Grok 3 Beta — The Age of Reasoning Agents - Grok Blog [Link]
Motivated by unmet needs in the modern scientific discovery process and building on recent AI advances, including the ability to synthesize across complex subjects and to perform long-term planning and reasoning, we developed an AI co-scientist system. The AI co-scientist is a multi-agent AI system that is intended to function as a collaborative tool for scientists. Built on Gemini 2.0, AI co-scientist is designed to mirror the reasoning process underpinning the scientific method. Beyond standard literature review, summarization and “deep research” tools, the AI co-scientist system is intended to uncover new, original knowledge and to formulate demonstrably novel research hypotheses and proposals, building upon prior evidence and tailored to specific research objectives.
― Accelerating scientific breakthroughs with an AI co-scientist - Google Blog [Link]
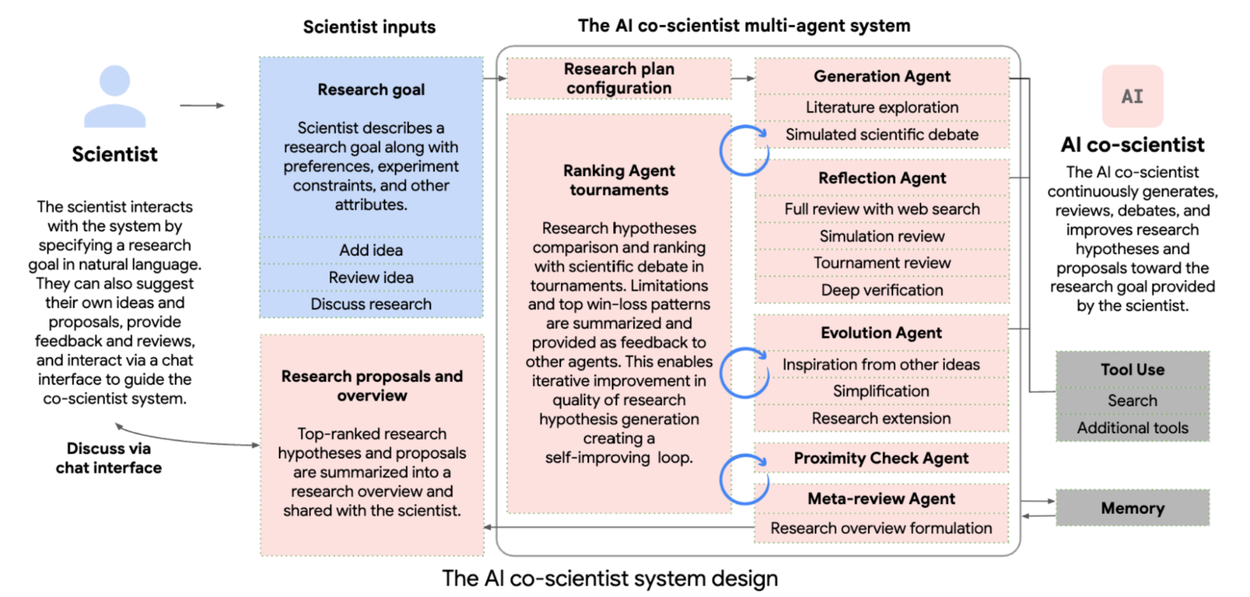
An Interview with Uber CEO Dara Khosrowshahi About Aggregation and Autonomy - Stratechery [Link]
OpenAI Deep Research Guide - DAIR.AI [Link]
This is a super helpful guide to Deep Research Tools.
You provide use cases and examples, very inspiring, and tips, etc.
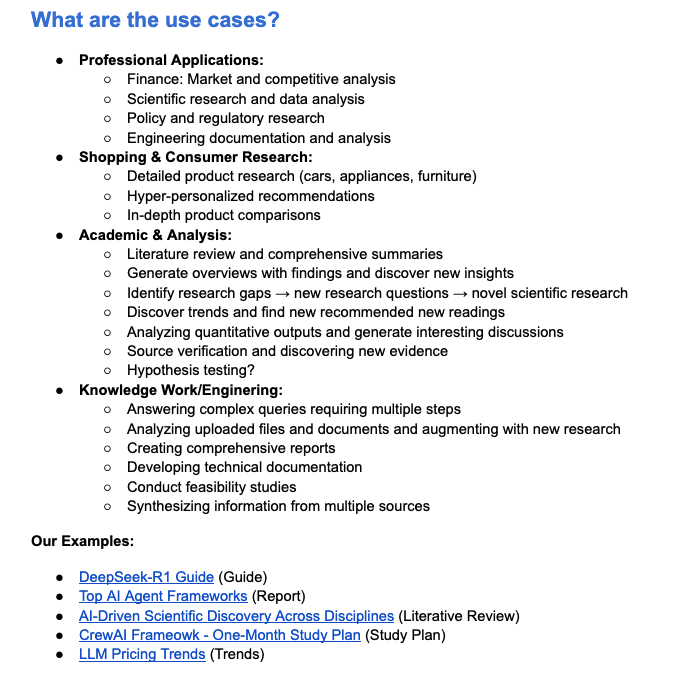
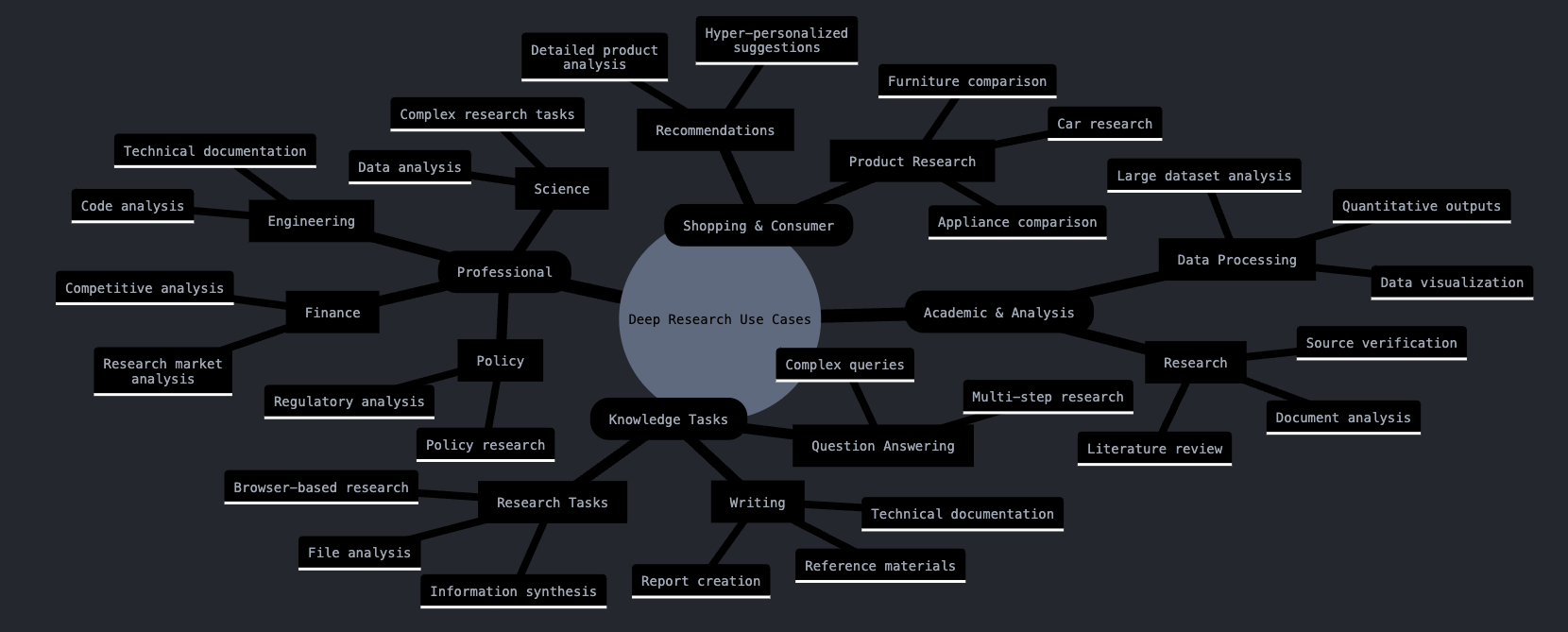
Studies on the brain affirm the benefits of Tom’s visualization technique: Imagining something in vivid detail can fire the same brain cells actually involved in doing that activity. The new brain circuitry appears to go through its paces, strengthening connections, even when we merely repeat the sequence in our minds. So to alleviate the fears associated with trying out riskier ways of leading, we should first visualize some likely scenarios. Doing so will make us feel less awkward when we actually put the new skills into practice.
― Primal Leadership: The Hidden Driver of Great Performance - Harvard Business Review [Link]
Imagine it, fake it, and make it.
Our research tells us that three conditions are essential to a group’s effectiveness: trust among members, a sense of group identity, and a sense of group efficacy.
― Building the Emotional Intelligence of Groups - Harvard Business Review [Link]
Team is so important to leaders.
Interrupt the ascent.
When people are continually promoted within their areas of expertise, they don’t have to stray far from their comfort zones, so they seldom need to ask for help, especially if they’re good problem solvers. Accordingly, they may become overly independent and fail to cultivate relationships with people who could be useful to them in the future. What’s more, they may rely on the authority that comes with rank rather than learning how to influence people. A command-and-control mentality may work in certain situations, particularly in lower to middle management, but it’s usually insufficient in more senior positions, when peer relationships are critical and success depends more on the ability to move hearts and minds than on the ability to develop business solutions.
― The Young and the Clueless - Harvard Business Review [Link]
Don't fall into the independence trap.
Accelerating scientific breakthroughs with an AI co-scientist - Google Research [Link]
News and Comments
Introducing deep research - Open AI [Link]
Introducing Perplexity Deep Research - Perplexity [Link]
Trend is on deep research.
Shopify Tells Employees to Just Say No to Meetings - Bloomberg [Link]
Who will control the future of AI? - The Washington Post [Link]
Sam promotes a U.S.-led strategy to ensure AI development aligns with democratic values and remains under the leadership of the U.S. and its allies.
This new architecture used to develop the Majorana 1 processor offers a clear path to fit a million qubits on a single chip that can fit in the palm of one’s hand.
Microsoft is now one of two companies to be invited to move to the final phase of DARPA’s Underexplored Systems for Utility-Scale Quantum Computing (US2QC) program – one of the programs that makes up DARPA’s larger Quantum Benchmarking Initiative – which aims to deliver the industry’s first utility-scale fault-tolerant quantum computer, or one whose computational value exceeds its costs.
― Microsoft’s Majorana 1 chip carves new path for quantum computing - Microsoft [Link]
In the near term, Google’s approach with superconducting qubits (like Willow) is more mature. This technology has already demonstrated impressive benchmarks and is backed by years of incremental improvements. Its error correction techniques, while still challenging, are well‑studied, and scaling up using transmon qubits is an area where significant progress has been made.
On the other hand, Microsoft’s topological approach with Majorana 1 aims to use a completely new type of qubit—one that is “protected by design” thanks to its topological nature. In theory, this means lower error rates and potentially a much more scalable architecture with fewer physical qubits needed per logical qubit. However, this method is still very experimental, and questions remain over whether true Majorana zero modes have been reliably created and controlled.
In summary, for near‑term practical applications, Google’s path appears to be the safer bet. But if Microsoft’s topological qubit platform can overcome its technical hurdles, it may ultimately provide a more efficient and scalable route to fault‑tolerant quantum computing.
Tencent's Weixin app, Baidu launch DeepSeek search testing - Reuters [Link]
OpenAI tries to ‘uncensor’ ChatGPT - Techcrunch [Link]
Elon Musk Ally Tells Staff ‘AI-First’ Is the Future of Key Government Agency - WIRED [Link]
Thomas Shedd, a former Tesla engineer and ally of Elon Musk, is implementing an "AI-first strategy" at the General Services Administration's Technology Transformation Services (TTS). Shedd envisions the agency operating like a software startup, automating tasks and centralizing federal data. This shift is causing concern among GSA staff, who report being thrown into unexpected meetings and facing potential workforce cuts. Shedd is promoting collaboration between TTS and the United States Digital Services (DOGE), though specifics about the new AI-driven projects and data repository remain unclear. A cybersecurity expert expressed concern that automating government tasks is difficult and the attempt is raising red flags. Employees also voiced concerns regarding working hours and potential job losses.
OpenAI o3-mini - OpenAI [Link]
Introducing deep research - OpenAI [Link]
Gemini 2.0 is now available to everyone - Google DeepMind [Link]
The all new le Chat: Your AI assistant for life and work - Mistral AI [Link]